A neuro-chip, also known as a neural implant, is a type of device that is designed to interface with the human brain in order to manage various brain disorders, such as epilepsy, Parkinson’s disease, depression, and other conditions that affect brain function. The neuro-chip is surgically implanted in the brain, where it can interact with the neural network and modulate the activity of specific brain regions.
For example, in the case of Parkinson’s disease, a neuro-chip can be used to deliver electrical stimulation to specific regions of the brain that are involved in controlling movement. This stimulation can help to alleviate the symptoms of Parkinson’s, such as tremors, stiffness, and difficulty with movement.
Researchers created a neural interface that can identify and suppress symptoms of various neurological disorders by combining low-power chip design, machine learning algorithms, and soft implantable electrodes.
Mahsa Shoaran of the School of Engineering’s Integrated Neurotechnologies Laboratory collaborated with Stéphanie Lacour of the Laboratory for Soft Bioelectronic Interfaces to create NeuralTree, a closed-loop neuromodulation system-on-chip that can detect and alleviate disease symptoms. The system can extract and classify a broad set of biomarkers from real patient data and animal models of disease in-vivo, resulting in a high degree of accuracy in symptom prediction, thanks to a 256-channel high-resolution sensing array and an energy-efficient machine learning processor.
NeuralTree benefits from a neural network’s accuracy and the hardware efficiency of a decision tree algorithm. This is the first time we’ve been able to integrate such a complex, yet energy-efficient, neural interface for binary classification tasks like seizure or tremor detection, as well as multi-class tasks like finger movement classification for neuroprosthetic applications.
Mahsa Shoaran
“NeuralTree benefits from a neural network’s accuracy and the hardware efficiency of a decision tree algorithm,” Shoaran says. “This is the first time we’ve been able to integrate such a complex, yet energy-efficient, neural interface for binary classification tasks like seizure or tremor detection, as well as multi-class tasks like finger movement classification for neuroprosthetic applications.”
Their findings were presented at the 2022 IEEE International Solid-State Circuits Conference and published in the IEEE Journal of Solid-State Circuits, the integrated circuits community’s flagship journal.
Efficiency, scalability, and versatility
NeuralTree functions by extracting neural biomarkers – patterns of electrical signals known to be associated with certain neurological disorders – from brain waves. It then classifies the signals and indicates whether they herald an impending epileptic seizure or Parkinsonian tremor, for example. If a symptom is detected, a neurostimulator – also located on the chip – is activated, sending an electrical pulse to block it.
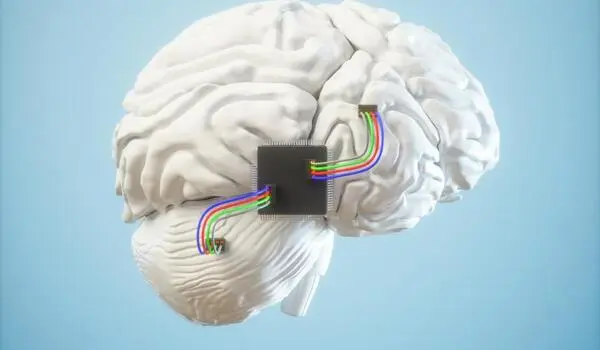
According to Shoaran, NeuralTree’s unique design provides the system with an unprecedented level of efficiency and versatility when compared to the state-of-the-art. The chip has 256 input channels, compared to 32 in previous machine-learning-embedded devices, allowing the implant to process more high-resolution data. Because of the chip’s area-efficient design, it is also extremely small (3.48mm2), providing great potential for scalability to more channels. NeuralTree is also highly energy efficient due to the incorporation of a ‘energy-aware’ learning algorithm, which penalizes features that consume a lot of power.
In addition to these benefits, the system can detect a broader range of symptoms than previous devices, which were primarily focused on epileptic seizure detection. The chip’s machine learning algorithm was trained on datasets from patients with epilepsy and Parkinson’s disease, and it correctly classified pre-recorded neural signals from both groups.
“This is, to the best of our knowledge, the first demonstration of Parkinsonian tremor detection using an on-chip classifier,” Shoaran says.
Self-updating algorithms
Shoaran is passionate about making neural interfaces more intelligent to enable more effective disease control, and she is already looking ahead to further innovations.
“We will eventually be able to use neural interfaces to treat a wide range of disorders, but we will need algorithmic ideas and advances in chip design to get there. Because this work is highly interdisciplinary, it also necessitates collaboration with labs such as the Laboratory for Soft Bioelectronic Interfaces, which can develop cutting-edge neural electrodes, or labs with access to high-quality patient data.”
She is interested in enabling on-chip algorithmic updates to keep up with the evolution of neural signals as a next step. “Because neural signals change over time, the performance of a neural interface will deteriorate. We are constantly working to improve algorithm accuracy and reliability, and one method would be to enable on-chip updates, or algorithms that can update themselves.”