Researchers from the University of Amsterdam, in collaboration with colleagues from the University of Queensland and the Norwegian Institute for Water Research, have developed a machine-learning strategy for assessing chemical toxicity.
They describe their approach in an article for the special issue “Data Science for Advancing Environmental Science, Engineering, and Technology” in Environmental Science & Technology. When compared to traditional ‘in silico’ assessments based on Quantitative Structure-Activity Relationship (QSAR) modeling, the models developed in this study can lead to significant improvements.
According to the researchers, using machine learning to assess the hazard of molecules can vastly improve both the safe-by-design development of new chemicals and the evaluation of existing chemicals. The importance of the latter is demonstrated by the fact that European and US chemical agencies have identified approximately 800,000 chemicals that have been developed over the years but for which little to no information about environmental fate or toxicity is available.
Because testing chemical fate and toxicity requires a significant amount of time, effort, and resources, modeling approaches are already being used to predict hazard indicators. The Quantitative Structure-Activity Relationship (QSAR) model, in particular, is frequently used to relate molecular features such as atomic arrangement and 3D structure to physicochemical properties and biological activity.
The Quantitative Structure-Activity Relationship (QSAR) model, in particular, is frequently used to relate molecular features such as atomic arrangement and 3D structure to physicochemical properties and biological activity.
Experts classify a molecule into categories based on modeling results (or measured data where available), such as those defined in the Globally Harmonized System of Classification and Labeling of Chemicals (GHS). Molecules in specific categories are then subjected to additional research, active monitoring, and, eventually, legislation.
However, this process has inherent drawbacks, much of which can be traced back to the limitations of the QSAR models. They are often based on very homogeneous training sets and assume a linear structure-activity relationship for making extrapolations. As a result, many chemicals are not well-represented by existing QSAR models and their uses can potentially lead to substantial prediction errors and misclassification of chemicals.
Skipping the QSAR prediction
Dr. Saer Samanipour and co-authors propose an alternative evaluation strategy that skips the QSAR prediction step entirely in their paper published in Environmental Science & Technology. Samanipour, an environmental analytical scientist at the Van ‘t Hoff Institute for Molecular Sciences at the University of Amsterdam, collaborated with Dr. Antonia Praetorius, an environmental chemist at the same university’s Institute for Biodiversity and Ecosystem Dynamics.
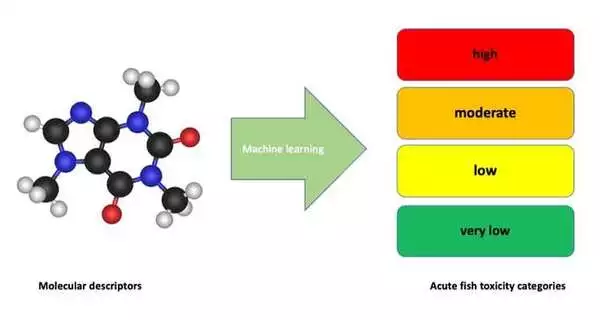
They developed a machine learning-based strategy for the direct classification of acute aquatic toxicity of chemicals based on molecular descriptors with colleagues from the University of Queensland and the Norwegian Institute for Water Research.
The model was developed and tested via 907 experimentally obtained data for acute fish toxicity (96h LC50 values). The new model skips the explicit prediction of a toxicity value (96h LC50) for each chemical, but directly classifies each chemical into a number of pre-defined toxicity categories. These categories can for example be defined by specific regulations or standardization systems, as demonstrated in the article with the GHS categories for acute aquatic hazard. The model explained around 90% of the variance in the data used in the training set and around 80% for the test set data.
Higher accuracy predictions
This direct classification strategy resulted in a fivefold decrease in the incorrect categorization compared to a strategy based on a QSAR regression model. Subsequently, the researchers expanded their strategy to predict the toxicity categories of a large set of 32,000 chemicals.
They show that their direct classification approach produces more accurate predictions because experimental datasets from various sources and chemical families can be grouped to generate larger training sets. It can be tailored to meet the requirements of various international regulations and classification or labeling systems. The direct classification approach has the potential to be expanded to other hazard categories (e.g., chronic toxicity) as well as environmental fate (e.g., mobility or persistence) in the future, and it shows great promise for improving in-silico tools for chemical hazard and risk assessment.