Researchers integrated multiple x-ray imaging methods to produce multi-contrast images capable of detecting dangerous objects such as explosives in thousands of complex scenarios. The novel approach, which also makes use of readily accessible machine learning procedures for materials classification, has the potential to be useful for security screening as well as applications in the life and physical sciences.
“This method is particularly well suited to discriminating objects with very similar elemental composition,” said research team leader Thomas Partridge from University College London in the UK. “It could be used in airport security or any inline scanning operation to examine materials flagged as suspicious by an initial fast scan such as a traditional x-ray system.”
In Optica, Optica Publishing Group’s journal for high-impact research, the researchers demonstrate that the new approach was highly effective in accurately detecting and identifying explosives in nearly 4,000 scans of threatening and non-threatening materials hidden inside bags or obscured by various types of objects. They attained a near-perfect recall rate of 99.68% for threat-bearing situations, with only one false negative.
“Although more work is needed, this approach could also prove useful for medical imaging,” Partridge added. “While traditional x-ray imaging struggles to separate healthy from diseased tissue, other studies have suggested that phase contrast imaging might be able to capture textures that could be used to distinguish healthy and benign tissues.”
This method is particularly well suited to discriminating objects with very similar elemental composition. It could be used in airport security or any inline scanning operation to examine materials flagged as suspicious by an initial fast scan such as a traditional x-ray system.
Thomas Partridge
Unlocking material secrets
The x-ray machines found in airports or medical facilities are based on x-ray attenuation, which images the reduction in intensity in x-rays after they pass through a material. The new technique creates multi-contrast images by combining conventional x-ray attenuation data at various x-ray energies with x-ray phase information, which consists of refraction and dark-field channels.
“Many explosives and common everyday items are composed of primarily carbon, hydrogen, nitrogen and oxygen, a similarity that makes them difficult to separate with x-ray attenuation alone,” said Partridge. “The additional channels offer significantly better enhancement of edges as well as textures and grains of materials, allowing the discrimination of objects with very similar elemental compositions.”
This work builds on previous efforts by the researchers to use multi-contrast x-ray phase enhanced imaging with machine learning approaches for threat detection with a smaller number of explosives and benign objects.
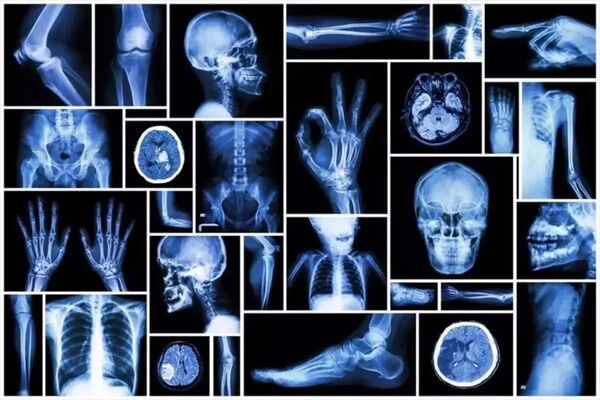
In the new experiment, they significantly scaled up the number of materials investigated and the number of imaging scenarios to better mimic real world situations. They also created a more effective scanning system with a resolution that could be changed by modifying the scanning speed and applied edge illumination phase contrast. Edge illumination involves placing masks before and after the sample to create the sub-pixel x-ray ‘beamlets’ needed to make the system sensitive to phase signals. One key advantage of this illumination approach is that it works with incoherent x-ray sources, widening its applicability.
Since the increased complexity of the imaging scenarios required more sophisticated protocols, the researchers applied machine learning with a hierarchical architecture that separated the cluttering objects before distinguishing material types. This made it possible to rapidly discern subtle differences in shapes and textures to distinguish materials based on key identifying features.
Detecting threats
To test the novel technique, they employed 19 threat materials and 56 non-threat materials, all at three thicknesses and disguised by a variety of cluttering objects such as brushes, face wipes, socks, and other items travelers would bring in a carry-on bag. Using all of the obtained contrast channels, the researchers demonstrated not just material discrimination, but also identification in some situations. Using deep learning to assess signals from a combination of x-ray contrasts yielded very encouraging results, with only one mistake out of 313 threat scenarios.
According to the researchers, adapting this strategy to a commercial context would necessitate increasing scanning speed through additional system optimization. The robustness of material discrimination should also be investigated on a bigger dataset. One active research area for the team is merging the approach with 3D computed tomography scanning, which is being investigated for security reasons due to its capacity to generate detailed, three-dimensional views of items.