Research papers come very often to anyone reading these. This is especially true of machine learning, which now applies to virtually every industry and organization (and creates paperwork). The purpose of this column is to collect some of the most relevant discoveries and documents – especially in artificial intelligence – but not limited to – and explains why they matter. We have lots of items with this version, AI or robotics and the interface between the real worlds.
Of course most of the applications of this type of technology have real-world applications but this study in particular is about the inevitable difficulties that occur due to the limitations of both sides of the real-virtual division. One issue that is constantly raised in robotics is how things slowly move in the real world. Naturally some robots trained on certain tasks can do them with superhuman speed and agility but in most cases this is not the case. Their observations against the virtual model of their world need to be tested so frequently that tasks like picking something up and putting it down can take minutes.
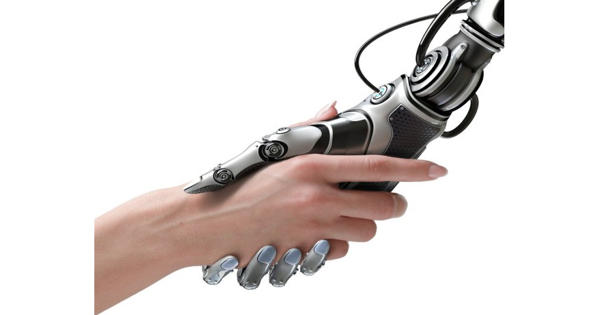
Particularly frustrating about this is that the real world is the best place to train robots, since in the end they will work on it. One way to address this is to increase the value of each hour of real-world testing you are doing, which is the goal of this project at Google. Rather in a technical blog post the team describes the challenge of learning and performing multiple tasks using and integrating data from multiple robots. It is complex, but they talked about creating an integrated process for assigning and evaluating tasks, and coordinating future assignments and assessments based on that.
More intuitively, they create a process through which success in Task A improves robots’ ability to perform Task B, even if they are different. People do it – for example learn how to throw a ball well. It’s important to create the most valuable training in the real-world and it shows a lot more optimization there. Another way is to improve the quality of simulations so that when robots take knowledge to the real world, they are closer to what will happen. This is the goal of the Allen Institute for AI’s tour training environment and its latest denizens, ManipulaTHOR.