An interdisciplinary team of researchers from the University of Missouri, Children’s Mercy Kansas City, and Texas Children’s Hospital employed a new data-driven strategy to learn more about Type 1 diabetes patients, who account for around 5-10% of all diabetes diagnoses. To better understand the condition, the researchers used health informatics to gather data and utilize artificial intelligence (AI).
The scientists reviewed publicly available, real-world data from over 16,000 people in the T1D Exchange Clinic Registry for the study. The team was able to uncover significant disparities in health outcomes among patients with Type 1 diabetes who have or do not have a direct family history of the condition by using a contrast pattern mining algorithm developed at the MU College of Engineering.
The AI strategy utilized in the study was directed by Chi-Ren Shyu, director of the MU Institute for Data Science and Informatics (MUIDSI), who described the technology as exploratory in nature.
“Here, we let the computer do the work of connecting millions of dots in the data to identify only major contrasting patterns between individuals with and without a family history of Type 1 diabetes, and we do the statistical testing to ensure we are confident in our results,” said Shyu, the Paul K. and Dianne Shumaker Professor in the MU College of Engineering.
Erin Tallon, a graduate student at MUIDSI and the study’s primary author, stated that the team’s investigation yielded some unexpected results.
Type 1 diabetes is not a single condition that looks the same for everyone; it looks different for various people. We’re working on the leading edge to solve that issue. By studying real-world data, we may gain a better understanding of the risk variables that put someone at a higher risk of developing bad health outcomes.
Erin Tallon
“For example, we discovered that those in the registry who had an immediate family member with Type 1 diabetes were more likely to have hypertension, as well as diabetes-related nerve illness, eye disease, and kidney disease,” Tallon explained. “We also discovered a higher frequency of co-occurrence of these diseases in people with a close family history of Type 1 diabetes. Individuals with an immediate family history of Type 1 diabetes were also more likely to have specific demographic traits.”
Tallon’s interest in this initiative began with a personal connection and swiftly evolved as a result of her experience working as a nurse in an acute critical care unit (ICU). She frequently saw patients with Type 1 diabetes who also had other co-existing diseases such as renal disease and excessive blood pressure. She intended to identify better strategies for prevention and diagnosis, starting with analyzing the huge quantities of publicly available data already collected about the disease, knowing that a person’s Type 1 diabetes diagnosis often comes only when the disease is already highly advanced.
Mark Clements, a pediatric endocrinologist at Children’s Mercy Kansas City, a professor of pediatrics at the University of Missouri-Kansas City, and the study’s corresponding author, was asked to talk at the Midwest Bioinformatics Conference hosted by BioNexus KC in 2019. Tallon was unable to attend Clements’ lecture, but she followed up with a phone call to convey her proposal for helping people understand Type 1 diabetes better. He was piqued. Tallon eventually introduced Clements to Shyu, and long-term research cooperation was created.
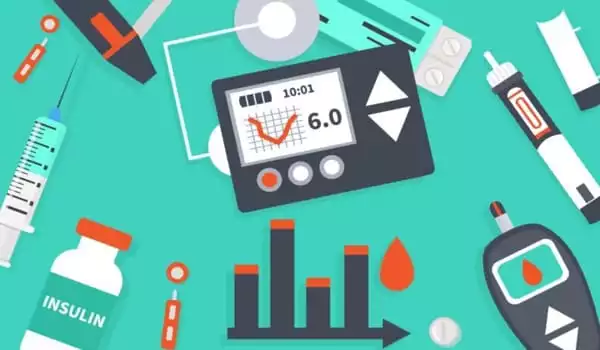
Tallon believes the collaboration’s outcomes demonstrate the potential and usefulness of leveraging real-world data. “Type 1 diabetes is not a single condition that looks the same for everyone; it looks different for various people,” Tallon explained. “We’re working on the leading edge to solve that issue.” “By studying real-world data, we may gain a better understanding of the risk variables that put someone at a higher risk of developing bad health outcomes.”
While the findings are encouraging, Tallon claims that the researchers were hampered by the lack of a population-based data collection to work with. “It is crucial to emphasize that our findings do have a drawback that we intend to overcome in the future by using larger, population-based data sets,” Tallon explained. “We want to construct larger patient cohorts and analyze more data, and we’re going to employ these algorithms to help us achieve that.”
Personalizing medicine
Clements expects that the concept will be embraced as a means of assisting in the development of individualized treatment choices for persons with diabetes.
“In order to get the right treatment to the right patient at the right time,” Clements explained, “we first need to understand how to identify patients who are at a higher risk for the disease and its complications — by asking questions like, “Are there characteristics early in someone’s life that can help identify an individual with high risk for an outcome year down the road?” “Having all of this information could one day help us construct a more full picture of a person’s risk, which we can utilize to develop a more individualized strategy to both prevention and therapy.”
“Contrast pattern mining with the T1D Exchange Clinic Registry indicates complicated phenotypic variables and comorbidity patterns associated with familial versus sporadic Type 1 diabetes,” the researchers wrote in Diabetes Care, an American Diabetes Association journal. The study was also supported by MU graduate students Danlu Liu and Katrina Boles, as well as Maria Redondo at Texas Children’s Hospital.
The authors would like to thank the T1D Exchange Clinic Registry’s funding agency, the Helmsley Charitable Trust, the investigators across the country who drove data collection for the registry, and all of the registry’s participants and their families who were willing to share their medical information.