Artificial intelligence (AI) is becoming more common in many industries, including online retailing. Traditional lines of work, such as transportation logistics and driving, are evolving in a similar manner, albeit mostly behind closed doors. Scientists from the University of Göttingen have now investigated how effective the use of artificial intelligence (AI) can be in the commercial management of trucks. Their response: an intelligent combination of human decision-making and AI applications is the best option. The findings of the study were published in the International Journal of Logistics Management.
“As in the private sector, digital applications – as well as machine learning, a type of AI – are increasingly permeating operations and processes in the transport and logistics sector,” explains Professor Matthias Klumpp of the Faculty of Economics. “The question in the commercial sector, however, is whether or not this contributes to the achievement of company goals and efficiency.”
Scientists have now investigated how efficient the use of AI is in the commercial management of trucks. Their answer: the best option is an intelligent combination of human decision-making and AI applications.
To answer this question, the researchers compared truck drivers’ work efficiency to their use of AI applications such as dynamic real-time navigation systems, cruise control, and automated gear-shifting based on speed and topography, among others. They studied three comparison groups when it came to retail trade delivery by truck: the first drove solely based on human decision-making patterns, the second used a combination of human and machine decision-making patterns, and the third relied solely on fully automated decisions.
Artificial intelligence will be used to improve the driver’s ability to control the vehicle in a variety of operating conditions. However, the amount of AI software and hardware required will far outnumber any computer system in today’s vehicles.
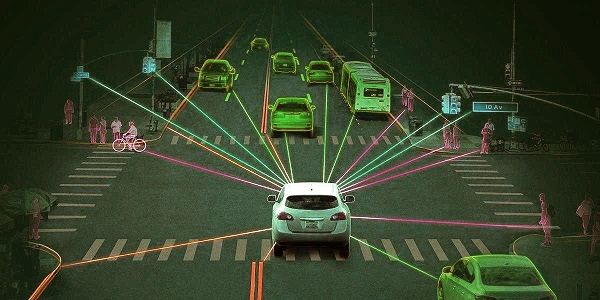
The Production and Logistics Research Group researchers concluded that an intelligent combination of human work and decision-making abilities with AI applications promises the highest transport and driving efficiency: “On average, the second group obtained the most efficient transportation trips, with the fewest interventions and deviations from the optimal path,” the authors wrote. “Clearly, neither a purely human decision-making structure nor a fully automated driving system can guarantee meeting current logistics requirements.”
As a result, the scientists conclude that despite the advancement of AI in the field of truck transportation, human experience, and decision-making capabilities will still be required in the long run. “However, working with AI applications will necessitate extensive training and qualification requirements, particularly for simple logistics activities,” the authors conclude. “As a result, technological and AI advancements are not solely the responsibility of management. Their application in operational transportation, in particular, can achieve efficiency and competitive advantages.”
The majority of AI hardware and software incorporates a variety of technologies. Deep learning, which mimics the activity of the brain’s ability to think, is particularly important. In a very real sense, this software learns to recognize patterns in digital representations of sounds, images, and other data.
Deep-learning technology, in fact, is expected to be one of the largest and fastest-growing areas of automotive AI. It’s used in voice recognition, voice search, recommendation engines, sentiment analysis, image recognition, and motion detection, among other things. Deep-learning technology is used in self-driving cars for image processing, speech recognition, and data analysis.
In recent years, both research and industry have paid close attention to self-driving cars. On the way to fully autonomous vehicles, we hope to provide a computer assistant to assist a human driver. We want to take existing systems to the next level by allowing for natural and targeted interaction based on events both inside and outside the car. In order to predict driver intentions, we use a dataset derived from real-world driving recordings in conjunction with machine learning techniques. This will assist the car’s artificial co-pilot in determining the most efficient solution for a given road situation.