Quantum entanglement is a physical phenomenon that occurs when a group of particles is generated, interacts, or shares spatial proximity in such a way that the quantum state of each particle in the group cannot be described independently of the state of the others, even if the particles are separated by a large distance. The topic of quantum entanglement is central to the difference between classical and quantum physics: entanglement is a key feature of quantum mechanics that classical mechanics lack.
New research has boosted the field of machine learning on quantum computers by removing a potential barrier to the practical implementation of quantum neural networks. While theorists previously believed that training a quantum neural network would require an exponentially large training set, the quantum No-Free-Lunch theorem developed by Los Alamos National Laboratory shows that quantum entanglement eliminates this exponential overhead.
“Our findings show that both big data and big entanglement are useful in quantum machine learning. Even better, entanglement leads to scalability, which eliminates the barrier of exponentially increasing the size of data in order to learn it” According to Andrew Sornborger, a computer scientist at Los Alamos and coauthor of the paper published in Physical Review Letters on February 18th. “The theorem gives us hope that quantum neural networks are on the right track toward quantum speed-up, and that they will eventually outperform their counterparts on classical computers.”
Our findings show that both big data and big entanglement are useful in quantum machine learning. Even better, entanglement leads to scalability, which eliminates the barrier of exponentially increasing the size of data in order to learn it.
Andrew Sornborger
Measurements of physical properties such as position, momentum, spin, and polarization performed on entangled particles can be found to be perfectly correlated in some cases. For example, if a pair of entangled particles is generated such that their total spin is known to be zero, and one particle is found to have clockwise spin on a first axis, the spin of the other particle, measured on the same axis, is found to be anticlockwise.
However, this behavior produces seemingly paradoxical effects: any measurement of a particle’s properties results in an irreversible wave function collapse of that particle, changing the original quantum state. When dealing with entangled particles, such measurements have an impact on the entire system.
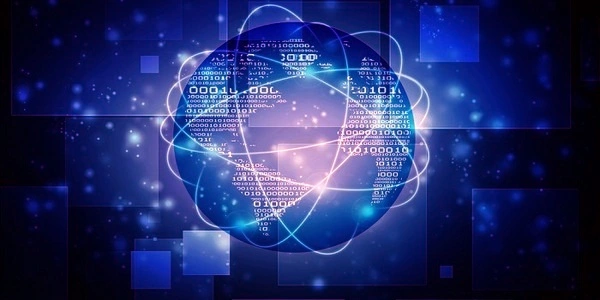
According to the classic No-Free-Lunch theorem, any machine-learning algorithm is as good as, but not better than, any other when their performance is averaged across all possible functions connecting the data to their labels. This theorem, which demonstrates the power of data in classical machine learning, has the direct consequence that the more data one has, the better the average performance. Thus, in machine learning, data is the currency that ultimately limits performance.
The new Los Alamos No-Free-Lunch theorem demonstrates that entanglement is also a currency in the quantum regime, and that it can be exchanged for data to reduce data requirements. The team used a Rigetti quantum computer to entangle the quantum data set with a reference system in order to validate the new theorem.
“We demonstrated on quantum hardware that we could effectively violate the standard No-Free-Lunch theorem using entanglement, while our new formulation of the theorem held up under experimental test,” said Kunal Sharma, the article’s first author.
“Our theorem suggests that, like big data, entanglement should be considered a valuable resource in quantum machine learning,” said Patrick Coles, a physicist at Los Alamos and senior author on the paper. “Classical neural networks rely solely on big data.”
Entanglement describes the state of a system of atomic-scale particles that cannot be fully described independently or individually. Entanglement is a critical component of quantum computing.
In quantum computers, changing the state of an entangled qubit immediately changes the state of the paired qubit. As a result, entanglement improves the processing speed of quantum computers. Doubling the number of qubits does not necessarily double the number of processes because processing one qubit reveals information about multiple qubits (i.e. the entangled qubits). According to research, quantum entanglement is required for a quantum algorithm to offer an exponential speedup over classical computations.