Version Space Learning is, which itself is a set – possibly infinite – containing all consistent hypotheses, can be represented by just its lower and upper bounds, and learning operations can be performed just on these representative sets. It is a logical approach to machine learning, specifically binary classification. Version Space framework is not a popular learning algorithm, there are some practical implementations that have been developed. A major drawback of version space learning is its inability to deal with noise: any pair of inconsistent examples can cause the version space to collapse, i.e., become empty, so that classification becomes impossible.
Version Space Learning
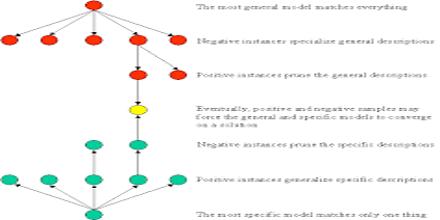