An experimental computing system physically modeled after the biological brain has “learned” to recognize handwritten numbers with a 93.4% overall accuracy. The experiment’s primary breakthrough was a new training method that provided the system with constant feedback on its task completion in real-time as it learned. The findings were reported in the journal Nature Communications.
The technique outperformed a traditional machine-learning strategy in which training was done after a batch of data was analyzed, achieving 91.4% accuracy. The researchers also demonstrated that storing past inputs in the system itself improved learning. Other computing systems, on the other hand, store memory in software or hardware independent of a device’s processor.
For the past 15 years, researchers at UCLA’s California NanoSystems Institute, or CNSI, have been working on a new computing platform technology. The technology is a brain-inspired system made out of a tangled web of silver-plated wires put on a bed of electrodes. The system uses electrical pulses to receive input and produce output. The diameter of the individual wires is measured on the nanoscale, in billionths of a meter.
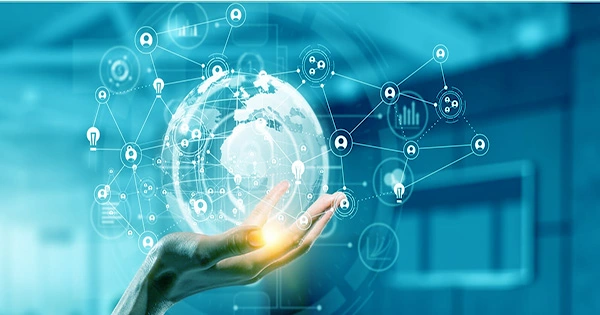
The “tiny silver brains” are not like today’s computers, which have distinct memory and processing units comprised of atoms whose locations do not change when electrons pass through them. The nanowire network, on the other hand, physically reconfigures in response to input, with memory based on its atomic structure and distributed across the system. Connections can form or break where wires overlap, similar to how synapses in the human brain communicate with one another.
Researchers at the University of Sydney created a streamlined algorithm for delivering input and understanding results. The algorithm is tailored to make use of the system’s brain-like ability to alter dynamically and process many streams of data at the same time.
The brain-like system was built of a silver and selenium-containing substance that was allowed to self-organize into a network of entangled nanowires on top of a grid of 16 electrodes.
The nanowire network was trained and evaluated using photographs of handwritten numerals from a dataset created by the National Institute of Standards and Technology and commonly used for benchmarking machine-learning systems. Images were conveyed to the system pixel by pixel using one-thousandth-second pulses of electricity, with varying voltages indicating light or dark pixels.
The nanowire network, which is still in development, is predicted to use significantly less power than silicon-based artificial intelligence systems to execute equivalent functions. The network also shows potential in jobs where existing AI struggles with making sense of complex data, such as weather patterns, traffic patterns, and other systems that vary over time. To accomplish so, today’s AI necessitates massive volumes of training data as well as extremely significant energy expenditures.
With the type of co-design utilized in this study—hardware and software created concurrently—nanowire networks could eventually serve as a complement to silicon-based electronic devices. Brain-like memory and processing incorporated in physical systems capable of constant adaptation and learning may be particularly well-suited to so-called “edge computing,” which processes complicated data on the fly without the need for connectivity with distant servers.
Potential applications include robotics, autonomous navigation in machines such as automobiles and drones, and the Internet of Things smart device technologies, as well as health monitoring and coordinating readings from sensors in many places.