According to new results by a team of Duke brain researchers, there are at least eight differences between a social butterfly and a lone wolf. The researchers discovered that by simultaneously monitoring the electrical activity of numerous brain regions, they could determine whether a mouse is gregarious or solitary. Then, by tinkering with nodes in this social brain network, they demonstrated that they could induce mice to be even more gregarious.
The findings could pave the way for better diagnostic tools to better understand how the brain changes in persons with impaired social communication, such as those with an autism spectrum disorder. The new study is published in the journal Neuron, which is available online.
Neuroscientists frequently conduct research by focusing on a single little brain region at a time. They may choose a favored brain area based on previous hints about its participation in a specific function, such as social behavior, and examine that area in isolation.
Looking at only one small brain region at a time, however, is a major issue, according to Dr. Kafui Dzirasa, Howard Hughes Medical Investigator, and K. Ranga Rama Krishnan Associate Professor of psychiatry and behavioral sciences at Duke. An automobile, like the brain, is made up of assembled elements that work together in different ways depending on the build, he says.
“An automobile does not have a steering wheel. An automobile is not made up of tires. An automobile is not a machine. The speedometer on an automobile is not the speedometer. The headlights of an automobile are not the same as the headlights of a car. To get the car, you must put them all together” Dzirasa stated this. “When you add them all up, you can calculate how quickly each individual car is travelling. Certainly, the tires could be the same, but a Lamborghini does not move like a Honda Accord. “
“What we’re attempting to do is create an AI system that can learn and describe what’s going on in the brain, and we’re attempting to approach this AI as a partner in the scientific process.
David Carlson
Dzirasa’s team first delicately implanted a recording device to capture the simultaneous electrical activity of eight brain regions that coordinate different aspects of social behavior, such as the prefrontal cortex and the dopamine-issuing ventral tegmental area, to figure out what drives mice to socialize.
The mice were then given the option of interacting with another mouse or a small stack of black Legos while the researchers monitored their brain waves. Surprisingly, no single electrical activity in the brain region could predict how social a mouse was during the test. Dzirasa, despite being a professional engineer, required assistance in compiling all of the intricate brain wave data.
“We [neuroscientists] have no idea how to make an automobile. We just see the wheels and tires. We don’t know how the system works “Dzirasa stated.
Dzirasa contacted his long-time associate David Carlson, an assistant professor of civil and environmental engineering, biostatistics, and bioinformatics, and a member of the Duke Institute for Brain Sciences, to assist in assembling a fuller picture of the data. Carlson is an expert in machine learning, a kind of artificial intelligence (AI) in which computers improve their comprehension of difficult material as they are given more of it. Carlson assisted in the development of a novel AI system to interpret brain wave data.
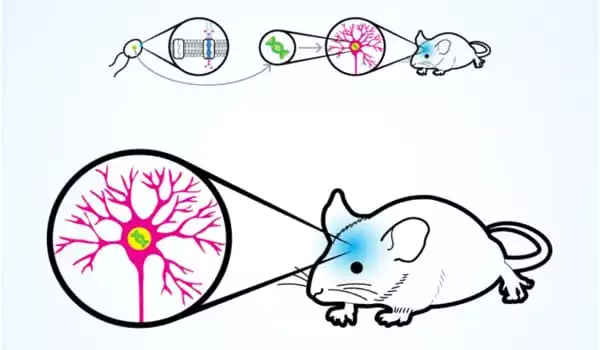
“What we’re attempting to do is create an AI system that can learn and describe what’s going on in the brain, and we’re attempting to approach this AI as a partner in the scientific process,” Carlson explained.
Importantly, the new AI tool can evaluate every electrical activity from every brain region, as well as tens of thousands of brain cells, and create a new “social brain network” map. Researchers can now determine which animals prefer the company of their peers based solely on the combined activity of their social brain network.
Dzirasa’s team examined if activating this social brain network would make mice even more social, armed with a new map and a crystal ball for social tendencies. To acquire precise control over particular brain regions, the researchers employed optogenetics, a light-based technology that allows them to switch on and off specific brain regions at a whim. Lighting up prefrontal cortex brain cells caused previously outgoing mice to become even more attached to another mouse, implying that this social brain network perceives and regulates social behavior.
Dzirasa then explored whether this social brain network model could detect poor social behavior in a mouse model of autism as a final test. When Dzirasa’s team took out ANK2, a gene associated with autism, in mice, their behavior perplexed the machine learning program. It could no longer predict how social a particular mouse was based on its brain waves, indicating that the new machine learning technology is capable of detecting abnormal electrical activity.
Dzirasa and Carlson are keen to see how these findings in mice translate to humans. Carlson’s group is currently working with researchers at the Duke Center for Autism and Brain Development to see if this new machine learning method can detect brain alterations in both typical and neurodiverse children.
Dzirasa seeks to improve diagnostic tools and treatments for different types of social problems. He compares this goal to cardiology: an EKG can monitor anyone’s active heart rate and compare it to their resting heart rate, which varies greatly between persons. However, there is no comparable technology for tracking and treating brain problems in the same way. There is no pacemaker to dial in the “correct” level of gregariousness, either, which is another future goal of Dzirasa’s study.
Dzirasa, on the other hand, is quick to emphasize that variance is normal, thus this study and future work are critical for understanding how things go wrong outside of someone’s regular range of sociability.
“Some people are more gregarious than others. It does not imply that persons who are less social suffer from a psychiatric disorder; rather, it indicates that there are individual variances” Dzirasa stated this. “If you want to eventually connect this to how persons are affected in psychiatric disease, you have to understand the individual.”