Based on data analysis, artificial intelligence (AI) has the potential to make predictions about various aspects of people’s lives. Artificial intelligence can analyze registry data on people’s residence, education, income, health, and working conditions and predict life events with high accuracy.
People’s lives can be predicted using artificial intelligence developed to model written language. A study conducted by DTU, the University of Copenhagen, ITU, and Northeastern University in the United States found that if large amounts of data about people’s lives are used to train so-called ‘transformer models,’ which (like ChatGPT) are used to process language, they can systematically organize the data and predict what will happen in a person’s life, as well as estimate the time of death.
Researchers used a model called life2vec to analyze health data and attachment to the labor market for 6 million Danes in a new scientific article titled ‘Using Sequences of Life-events to Predict Human Lives’, which was published in Nature Computational Science. After the model has been trained, or learned the patterns in the data, it has been shown to outperform other advanced neural networks (see fact box) and accurately predict outcomes such as personality and time of death.
“We used the model to address the fundamental question: to what extent can we predict events in your future based on conditions and events in your past? Scientifically, what is exciting for us is not so much the prediction itself, but the aspects of data that enable the model to provide such precise answers,” says Sune Lehmann, professor at DTU and first author of the article.
The model opens up important positive and negative perspectives to discuss and address politically. Similar technologies for predicting life events and human behavior are already used today inside tech companies that, for example, track our behavior on social networks, profile us extremely accurately, and use these profiles to predict our behavior and influence us.
Sune Lehmann
Predictions of time of death
Life2vec’s predictions are responses to general questions such as “death within four years?” When the researchers analyze the model’s responses, the results are consistent with existing findings in the social sciences; for example, all else being equal, individuals in a leadership position or with a high income are more likely to survive, whereas being male, skilled, or having a mental diagnosis is associated with a higher risk of dying.
Life2vec encodes the data in a large system of vectors, a mathematical structure that organizes the various data. The model determines where to place data on birth date, schooling, education, salary, housing, and health.
“What’s exciting is to consider human life as a long sequence of events, similar to how a sentence in a language consists of a series of words. This is usually the type of task for which transformer models in AI are used, but in our experiments, we use them to analyze what we call life sequences, i.e., events that have happened in human life,” says Sune Lehmann.
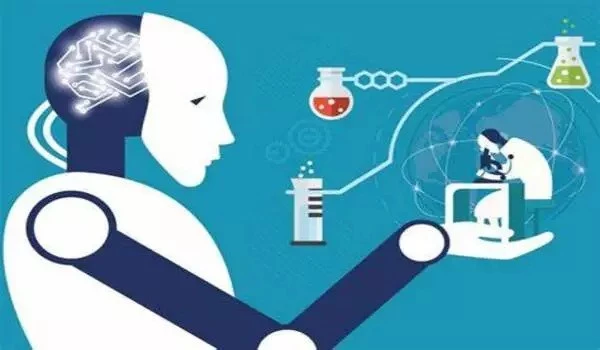
Raising ethical questions
The researchers behind the article point out that the life2vec model raises ethical concerns, such as protecting sensitive data, privacy, and the role of bias in data. Before the model can be used to assess an individual’s risk of contracting a disease or other preventable life events, these challenges must be thoroughly understood.
“The model opens up important positive and negative perspectives to discuss and address politically. Similar technologies for predicting life events and human behavior are already used today inside tech companies that, for example, track our behavior on social networks, profile us extremely accurately, and use these profiles to predict our behavior and influence us. This discussion needs to be part of the democratic conversation so that we consider where technology is taking us and whether this is a development we want,” says Sune Lehmann.
According to the researchers, the next step would be to incorporate other types of information, such as text and images or information about our social connections. This use of data opens up a whole new interaction between social and health sciences.
The research project
The ‘Using Sequences of Life-Events to Predict Human Lives’ research project is based on labor market data as well as data from the National Patient Registry (LPR) and Statistics Denmark. The dataset includes information on all 6 million Danes, including income, salary, stipend, job type, industry, social benefits, and so on. The health dataset contains information about visits to healthcare professionals or hospitals, as well as diagnosis, patient type, and level of urgency. The dataset spans from 2008 to 2020, but researchers focus on the 2008-2016 period and an age-restricted subset of individuals in several analyses.
Transformer model
A transformer model is a deep learning data architecture that uses artificial intelligence to learn about language and other tasks. Language understanding and generation models can be trained. The transformer model, which is often used to train large language models on large datasets, is designed to be faster and more efficient than previous models.
Neural networks
A neural network is a computer model inspired by the human and animal brain and nervous systems. There are numerous types of neural networks (for example, transformer models). A neural network, like the brain, is made up of artificial neurons. These neurons are linked and can communicate with one another. Each neuron receives input from other neurons and then computes an output that is then passed on to other neurons. By training on large amounts of data, a neural network can learn to solve tasks. Training data is used by neural networks to learn and improve their accuracy over time. However, once these learning algorithms have been fine-tuned for accuracy, they become powerful tools in computer science and artificial intelligence, allowing us to classify and group data at high speed. Google’s search algorithm is one of the most well-known neural networks.