The machine-learning approach accurately assesses risk factors and predicts the eventual development of conduct disorder. Elsevier publishes the work in Biological Psychiatry: Cognitive Neuroscience and Neuroimaging.
CD is a common yet complex psychiatric illness characterized by aggressive and destructive conduct. The factors that contribute to the development of CD are biological, psychological, and social in nature. Researchers have identified a plethora of risk variables that may aid in the prediction of CD, although they are frequently considered in isolation. For the first time, a new study employs a machine-learning approach to analyze risk variables across all three domains in tandem and predict the eventual development of CD with excellent accuracy.
The findings are published in Elsevier’s Biological Psychiatry: Cognitive Neuroscience and Neuroimaging. Oppositional defiant disorder (ODD) is a childhood behavior disorder characterized by children’s uncooperative, confrontational, and argumentative behavior in order to anger and annoy those in charge.
Our findings show the added utility of combining neurological, social, and psychological characteristics to predict conduct disorder, a severe psychiatric disease in kids. These findings hold promise for creating more precise diagnostic and therapeutic strategies that take into account the numerous aspects that contribute to this illness.
Arielle Baskin-Sommers
The researchers analyzed baseline data from almost 2,300 adolescents aged 9 to 10 who participated in the Adolescent Brain Cognitive Development (ABCD) Study, a longitudinal study that tracks children’s biopsychosocial development. The researchers used previously discovered risk factors from numerous biopsychosocial domains to “train” their machine-learning model. Measures included, for example, brain imaging (biological), cognitive abilities (psychological), and familial traits (social). With greater than 90% accuracy, the model anticipated the appearance of CD two years later.
Cameron Carter, MD, Editor of Biological Psychiatry: Cognitive Neuroscience and Neuroimaging, said of the study: “These striking results using task-based functional MRI to investigate the function of the reward system suggest that risk for later depression in children of depressed mothers may depend more on mothers’ responses to their children’s emotional behavior than on the mother’s mood per se.”
The ability to reliably identify who will develop CD would help researchers and healthcare workers devise interventions for at-risk youngsters, with the potential to reduce or perhaps avoid the detrimental impacts of CD on children and their families.
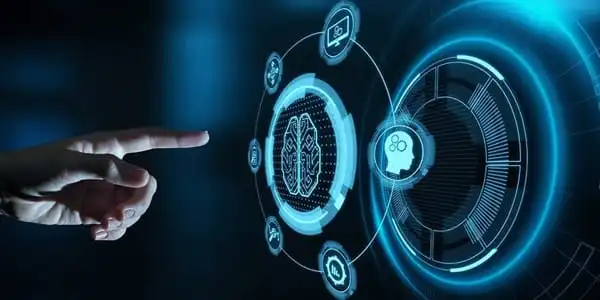
“Our findings show the added utility of combining neurological, social, and psychological characteristics to predict conduct disorder, a severe psychiatric disease in kids,” said senior author Arielle Baskin-Sommers, PhD, of Yale University in New Haven, Connecticut. “These findings hold promise for creating more precise diagnostic and therapeutic strategies that take into account the numerous aspects that contribute to this illness. They also emphasize the value of using huge, open-access datasets, such as ABCD, which capture measures about individuals at multiple levels of analysis.”
Machine learning techniques are gaining traction in neuroimaging applications for analysis and prediction. Hand-engineered feature selection is required for traditional machine learning techniques, which are time-consuming and prone to bias due to manual feature selection. Deep learning is a new advancement in machine learning that eliminates the problems associated with hand-engineering and the required domain expertise for feature selection.
Deep learning is a type of representation learning in which raw data is fed into a learning system that decomposes the input data into numerous levels of complicated nonlinear representative patterns. The adoption of a convolutional neural network (CNN) in 2012 reduced the second-best error rate for picture classification by about half at the ImageNet Large-Scale Visual Recognition Challenge, which can be linked to the blooming extensive applications of deep learning models.
Deep representation learning has been effectively used in a variety of disciplines, including image processing and analysis tasks, natural language processing, speech recognition, and data synthesis and analysis, since the advent of parallel computers and graphics processing units. Deep learning algorithms are increasingly being used in a vast range of medical imaging analyses to extract characteristics from raw data for further analysis and interpretation.