Researchers employed physics and machine learning to build a novel 3D printing technology that can swiftly construct complicated physical patterns, such as reproducing a part of a Pollock painting, by utilizing the same natural fluid instability that Pollock used in his work.
Can a machine be taught to paint in the style of Jackson Pollock? Can 3D printing, in particular, use Pollock’s distinct skills to swiftly and precisely produce complicated shapes?
“I wanted to know if it was possible to replicate Jackson Pollock and reverse engineer what he did,” said L. Mahadevan, the Lola England de Valpine Professor of Applied Mathematics at Harvard’s John A. Paulson School of Engineering and Applied Sciences (SEAS), as well as a Professor of Organismic and Evolutionary Biology and Physics in the Faculty of Arts and Sciences (FAS).
Mahadevan and his colleagues employed physics and machine learning to design a novel 3D-printing technology that can swiftly construct complicated physical patterns, such as reproducing a section of a Pollock painting, by harnessing the same natural fluid instability that Pollock used in his work.
We wanted to develop a technique that could take advantage of the folding and coiling instabilities, rather than avoid them.
Gaurav Chaudhary
The research is published in Soft Matter. 3D and 4D printing has revolutionized manufacturing but the process is still painstakingly slow.
The problem, as is customary, is physics. Because liquid inks follow the rules of fluid dynamics, when they fall from a great height, they become unstable, folding and coiling in on themselves. You may test this at home by sprinkling honey on some toast.
Mahadevan presented a simple scientific explanation of this process more than two decades ago, and later proposed how Pollock could have instinctively employed these principles to paint from a distance.
Most 3D and 4D printing systems now set the print nozzle millimeters from the surface, thereby removing the liquid stream’s dynamic instability. But Mahadevan has a motto: use physics rather than avoid it.
“We wanted to develop a technique that could take advantage of the folding and coiling instabilities, rather than avoid them,” said Gaurav Chaudhary, a former postdoctoral fellow at SEAS and the first author of the paper.
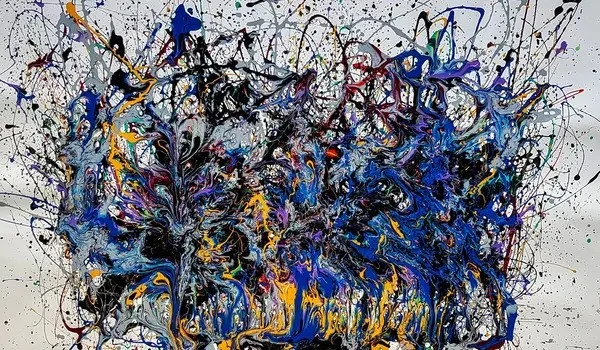
Pollock composed his famous drip paintings by placing a canvas on the floor and drizzling, pouring, dripping and splashing paint onto it from above. To the untrained eye, his technique may seem haphazard, but Pollock always claimed he had complete control over the flow of the paint.
Pollock, known for his “action painting,” drew in the space above the canvas, creating shapes in the air that would fall to the canvas below.
“If you look at traditional 3D printers, you supply them a path from point A to point B and the nozzle deposits ink along that specified path,” said Chaudhary, a software engineer. “However, Pollock’s method of throwing paint from a height meant that even if his hand was moving in a specific trajectory, the paint did not follow that trajectory due to gravity’s acceleration.” A tiny movement could cause a massive spatter of paint. Because of the free acceleration provided by gravity, you can print longer lengths than you can travel.”
The question was, how to control it?
To learn how to manipulate the nozzle to print at a distance and control fluid coiling, Mahadevan and Chaudhary, along with co-authors Stephanie Christ, a former student in Mahadevan’s Soft Math Lab and A. John Hart, Professor of Mechanical Engineering at MIT, combined the physics of coiling with deep reinforcement learning, which is an algorithmic approach to improving performance iteratively. Mahadevan and his team used techniques developed by Petros Koumoutsakos, Herbert S. Winokur, Jr. Professor of Computing in Science and Engineering at SEAS.
“With deep reinforcement learning, the model can learn from its mistakes and get more and more accurate with each trial,” Chaudhary said in a statement.
The researchers used this approach to print a succession of intricate forms, painting like Pollock and even adorning a biscuit with chocolate syrup. For this study, the researchers used simple fluids, but the approach might be broadened to incorporate more complicated fluids such as liquid polymers, pastes, and various sorts of meals.
“Using physical processes to achieve functional results is both a hallmark of intelligent behavior and at the heart of engineering design. This small example suggests that understanding the evolution of the first may help us be better at the second,” Mahadevan added.
As the research continues, there is no telling where Mahadevan may look for inspiration next.