The rise of many deadly diseases, such as infections caused by multidrug-resistant bacteria, necessitates re-inventing the drug discovery wheel. A better understanding of disease metabolism and regulation, advances in knowledge based on the genomes of disease-born microorganisms, the development of more representative disease models, and advancements in techniques, technologies, and computation applied to biology are all advances that will aid in drug discovery in the coming years.
Queen’s University Belfast researchers have created a computer-aided data tool that could improve treatment for a variety of illnesses. The computer modeling tool will predict novel binding sites for potentially more selective drugs, resulting in more effective drug targeting, increased therapeutic efficacy, and reduced side effects.
The data tool or protocol will lead to the discovery of a new class of compounds: allosteric drugs in G protein-coupled receptors (GPCRs). GPCRs are the most numerous membrane protein families that transduce signals from hormones, neurotransmitters, and other endogenous molecules inside cells. GPCRs are drug targets in many therapeutic areas due to their broad influence on human physiology, including inflammation, infertility, metabolic and neurological disorders, viral infections, and cancer. Currently, GPCRs are involved in the action of more than one-third of all drugs. Despite the significant therapeutic success, the discovery of GPCR drugs is difficult due to promiscuous binding and the resulting side effects.
We created a novel, cost-effective, and fast pipeline for identifying GPCR allosteric sites that overcomes the limitations of current computational protocols such as membrane distortion and non-specific binding. Our pipeline can identify allosteric sites quickly, making it suitable for industrial settings.
Dr. Irina Tikhonova
Recent research indicates the existence of additional binding sites, known as allosteric sites, to which drugs can bind and provide a variety of therapeutic benefits. Allosteric sites and drugs, on the other hand, have mostly been discovered by chance. Recent advances in X-ray crystallography, which determines the atomic and molecular structure, and cryo-electron microscopy, which provides 3D models of several GPCRs, provide opportunities to develop computer-aided methodologies for searching for allosteric sites.
The researchers created a computer-aided protocol to map allosteric sites in GPCRs in order to begin the rational search for allosteric drugs, which could lead to new solutions and therapies for a variety of diseases.
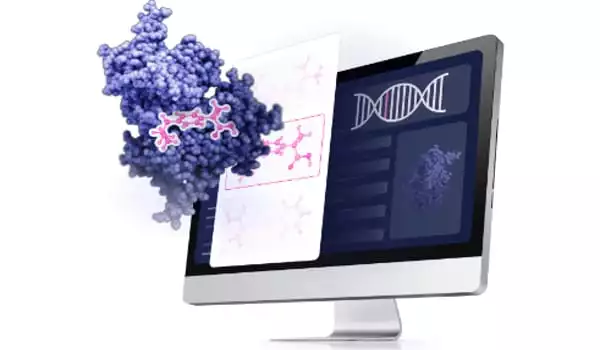
Dr. Irina Tikhonova, the senior author, and professor at Queen’s University’s School of Pharmacy explains: “We created a novel, cost-effective, and fast pipeline for identifying GPCR allosteric sites that overcomes the limitations of current computational protocols such as membrane distortion and non-specific binding. Our pipeline can identify allosteric sites quickly, making it suitable for industrial settings. As a result, our pipeline is a viable solution for starting the structure-based search for allosteric drugs for any membrane-bound drug targets with an impact on cancer, inflammation, or CNS diseases.”
In the pharmaceutical industry, there has been a significant increase in data digitization over the last few years. However, the challenge of acquiring, scrutinizing, and applying knowledge to solve complex clinical problems comes with digitalization. This encourages the use of AI, which can handle large amounts of data with enhanced automation. AI is a technology-based system that employs a variety of advanced tools and networks to simulate human intelligence. At the same time, it does not threaten to completely replace human physical presence. AI employs systems and software that can interpret and learn from input data in order to make independent decisions for achieving specific goals.
AI encompasses several method domains, including reasoning, knowledge representation, and solution search, as well as a fundamental machine learning paradigm (ML). ML employs algorithms that can recognize patterns in a previously classified set of data. Deep learning (DL) is a subfield of machine learning that employs artificial neural networks (ANNs). These are a collection of interconnected, sophisticated computing elements that include ‘perceptions,’ which are analogous to human biological neurons and mimic the transmission of electrical impulses in the human brain.