Despite our incredible advancements in science and healthcare, we have yet to find a treatment for cancer. On the plus side, we’ve made significant progress in identifying tumors in their early stages, allowing doctors to prescribe therapies that improve long-term survival.
This might be attributed to “integrated diagnosis,” a patient-care method that integrates genomic data and medical imaging data to detect cancer kind and, eventually, predict treatment results.
There are, however, a number of complexities. Radiogenomics is the study of the relationship between molecular patterns like gene expression and mutation and imaging aspects like how a tumor appears on a CT scan. The frequent usage of high-dimensional data, in which the number of features exceeds the number of observations, limits this discipline.
The ability to interrogate the model is critical to understanding and validating the learned radiogenomic associations.
William Hsu
Gene expression is a strictly controlled process that allows a cell to respond to changes in its surroundings. It serves as an on/off switch for controlling when proteins are created as well as a volume control for increasing or decreasing the number of proteins produced.
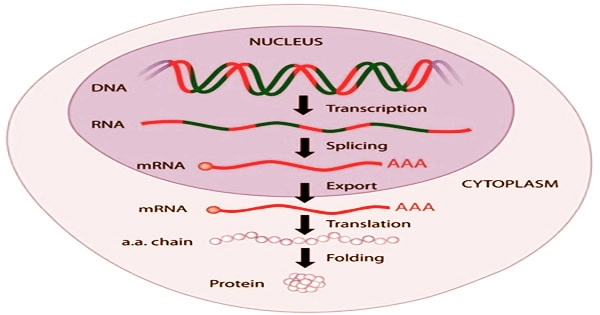
A paucity of validation datasets and various simple model assumptions plague radiogenomics. While deep neural networks and other machine learning approaches can help by accurately predicting visual characteristics from gene expression patterns, there is a new problem: we don’t know what the model has learnt.
“The ability to interrogate the model is critical to understanding and validating the learned radiogenomic associations,” explains William Hsu, associate professor of radiological sciences at the University of California, Los Angeles, and director of the Integrated Diagnostics Shared Resource. Hsu’s lab focuses on data integration, machine learning, and imaging informatics issues.
Hsu and his colleagues employed “gene masking,” a way of reading a neural network, to examine trained neural networks to discover learnt correlations between genes and imaging phenotypes in previous research. They showed that the radiogenomic connections found by their model matched previous information.
However, in their prior work, they only utilized a single dataset for brain tumors, therefore the generalizability of their technique was unknown.
In light of this, Hsu and his colleagues, Nova Smedley, a former graduate student and lead author, and Denise Aberle, a thoracic radiologist, conducted a study to see if deep neural networks can represent relationships between gene expression, histology (microscopic features of biological tissues), and CT-derived image features.
They discovered that the network could not only replicate but also discover previously unknown relationships. This study’s findings were reported in the Journal of Medical Imaging.
The researchers trained their neural networks on a dataset of 262 patients to predict 101 characteristics from a huge array of 21,766 gene expressions. They then put it to the test on an independent dataset of 89 patients, comparing it against the performance of other models in the training dataset.
Finally, they used gene masking to figure out which groups of genes were linked to which form of lung cancer. They discovered that neural networks performed better than other models in capturing these datasets and were generalizable to datasets from a different population.
Furthermore, the results of gene masking revealed that each imaging feature’s prediction was linked to a distinct gene expression profile driven by biological processes. The discoveries have given the researchers hope.
“While radiogenomic associations have previously been shown to accurately risk stratify patients, we are excited by the prospect that our model can better identify and understand the significance of these associations. We hope this approach increases the radiologist’s confidence in assessing the type of lung cancer seen on a CT scan. This information would be highly beneficial in informing individualized treatment planning,” observes Hsu.