Using 18th-century mathematics, researchers from the University of Jyväskylä were able to simplify deep learning, the most common artificial intelligence technique. They also discovered that classical training algorithms dating back 50 years outperform more recently popular approaches. Their straightforward approach promotes green IT while being easy to use and comprehend.
The recent success of artificial intelligence is largely due to the application of a single basic technique: deep learning. Deep learning refers to artificial intelligence approaches that use big datasets and a great amount of computer resources to train networks with a large number of data processing layers.
Deep learning enables computers to perform complex tasks such as analyzing and generating images and music, playing digitized games, and, most recently, acting as a natural language conversational agent that provides high-quality summaries of existing knowledge in conjunction with ChatGPT and other generative AI techniques.
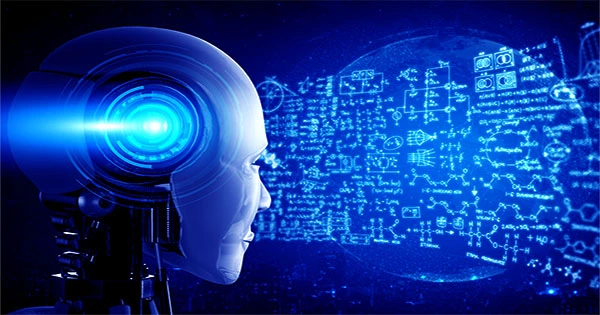
Professor Tommi Kärkkäinen and Doctoral researcher Jan Hänninen did early data reduction investigations six years ago. The findings were unexpected: depth is not required when combining simple network architectures in creative ways. Shallow models can produce comparable or even superior outcomes.
“The use of deep learning techniques is a complex and error-prone endeavor, and the resulting models are difficult to maintain and interpret,” Kärkkäinen said. “Our new model in its shallow form is more expressive and can reliably reduce large datasets while maintaining all the necessary information in them.”
The new AI technique’s structure can be traced back to 18th-century mathematics. Kärkkäinen and Hänninen also discovered that traditional optimization strategies from the 1970s function better in preparing their model than 21st-century deep learning techniques.
“Our results ensure that the use of neural networks in various applications is easier and more reliable than before,” Hänninen said. The findings have been published in the journal Neurocomputing.
Simpler models lead to greener and more ethical AI: Because artificial intelligence is playing an increasingly crucial role in current technologies, it is becoming increasingly vital to grasp how AI works.
“The more transparent and simpler AI is, the easier it is to consider its ethical use,” Kärkkäinen said. “For instance, in medical applications deep learning techniques are so complex that their straightforward use can jeopardize patient safety due to unexpected, hidden behavior.”
According to the researchers, simpler models can aid in the development of green IT and are more ecologically friendly because they conserve computational resources and use much less energy.
The findings, which used deep learning techniques to question common ideas and current public impressions, were difficult to disseminate.
“Deep learning has such a prominent role in research, development, and the AI business that, even if science always progresses and reflects the latest evidence, the community itself may have resistance to change.”
“We are very interested to see how these results will be received in the scientific and business community,” Kärkkäinen said. “Our new AI has a range of applications in our own research, from nanotechnology for better materials in the sustainable economy to improving digital learning environments and increasing the reliability and transparency of medical and well-being technology.”