Emu, an algorithm developed by computer scientists, uses long reads of genomes to identify the species of bacteria in a community. The program could make it easier to distinguish between harmful and beneficial bacteria in microbiomes such as those found in the gut, as well as in agriculture and the environment.
When it comes to identifying a microbe species, any part of a gene is better than none. However, part was not nearly enough for Rice University computer scientists in their pursuit of a program that could identify all the species in a microbiome. Emu, their microbial community profiling software, successfully identifies bacterial species by utilizing long DNA sequences that span the entire length of the gene under investigation.
The Emu project, led by computer scientist Todd Treangen and graduate student Kristen Curry of Rice’s George R. Brown School of Engineering, facilitates the analysis of a key gene microbiome researchers use to sort out bacteria species that may be harmful – or beneficial – to humans and the environment.
Their target, 16S, is a subunit of the rRNA (ribosomal ribonucleic acid) gene, which Carl Woese first used in 1977. This region is highly conserved in bacteria and archaea, and it also contains variable regions that are important for distinguishing between different genera and species.
While error rates have decreased in recent years, they can still have up to 10% error inside an individual DNA sequence, while species can be distinguished by a handful of differences in their 16S gene. The main computational challenge of this research project was distinguishing sequencing error from true differences.
Todd Treangen
“It’s commonly used for microbiome analysis because it’s found in all bacteria and most archaea,” Curry, a third-year member of the Treangen group, explained. “As a result, there are areas that have been preserved over time that are easy to target. We need parts of the DNA sequence to be the same in all bacteria so we know what to look for, and then we need parts to be different so we can tell bacteria apart.”
The Rice team’s study appears in the journal Nature Methods, along with collaborators from Germany, the Houston Methodist Research Institute, Baylor College of Medicine, and Texas Children’s Hospital.
“Years ago we tended to focus on bad bacteria — or what we thought was bad — and we didn’t really care about the others,” Curry said. “But there’s been a shift in the last 20 years to where we think maybe some of those other bacteria hanging out mean something.
“That is what we call the microbiome, all of the microscopic organisms in an environment,” she explained. “Water, soil, and the intestinal tract are commonly studied environments, and microbes have been shown to affect crops, carbon sequestration, and human health.”
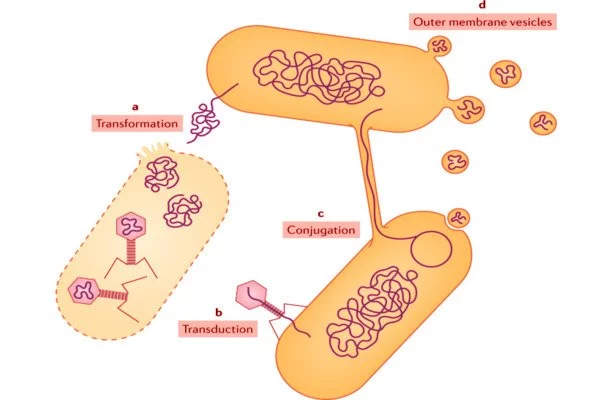
Emu, which derives its name from the task of “expectation maximization,” analyzes full-length 16S sequences from bacteria processed by an Oxford Nanopore MinION handheld sequencer and employs sophisticated error correction to identify species based on nine distinct “hypervariable regions.”
“Previously, we could only read a portion of the 16S gene,” Curry explained. “It has approximately 1,500 base pairs, and short-read sequencing can only sequence up to 25% -30% of this gene.” To achieve species-level precision, however, the full-length gene is required.”
However, even the most advanced technology isn’t perfect, allowing errors to creep into sequences.
“While error rates have decreased in recent years, they can still have up to 10% error inside an individual DNA sequence, while species can be distinguished by a handful of differences in their 16S gene,” said Treangen, an assistant professor of computer science who specializes in infectious disease tracking. “The main computational challenge of this research project was distinguishing sequencing error from true differences.”
“One issue is that a lot of the error is nonrandom, meaning it can occur repeatedly in specific positions, and then start to look like true differences instead of sequencing error,” he said.
“Another issue is there can be thousands of bacterial species in a given sample, creating a complex mixture of microbes that can exist at abundances well below the sequencing error rate,” Treangen said. “This means we can’t simply rely on ad hoc cutoffs to distinguish signal from error.”
Emu instead learns to distinguish between signal and error by comparing a large number of long sequences, first against a template and then against each other, iteratively improving its error-correction as it profiles microbial communities. In the experiments, false positives dropped significantly in Emu when compared to other approaches when analyzing the same data sets.
“Long-reads are a disruptive technology for microbiome research,” Treangen said. “Emu’s goal was to use all of the information contained in the full-length 16S gene without masking anything to see if we could make more accurate genus- or species-level calls. And that’s exactly what we did with Emu, thanks to a fruitful multidisciplinary collaborative effort.”