Professor Giuseppe Carleo of the Ecole Polytechnique Fédérale de Lausanne (EPFL) and Matija Medvidović, a graduate student at Columbia University and the Flatiron Institute in New York, have discovered a way to execute a complex quantum computing algorithm on traditional computers rather than quantum ones in a paper published in Nature Quantum Information.
Quantum computers are data storage and computing machines that take advantage of quantum physics characteristics. This may be highly beneficial for some jobs, where they can substantially outperform even our most powerful supercomputers. Traditional computers, such as smartphones and laptops, store data in binary “bits” that can be either 0s or 1s. A quantum bit, or qubit, is the fundamental memory unit of a quantum computer.
The Quantum Approximate Optimization Algorithm (QAOA) is a type of “quantum software” that is used to solve conventional optimization issues in mathematics. It is simply a method of selecting the best solution to a problem from a group of alternatives. “There is a lot of interest in understanding what problems can be solved efficiently by a quantum computer, and QAOA is one of the more prominent candidates,” says Carleo.
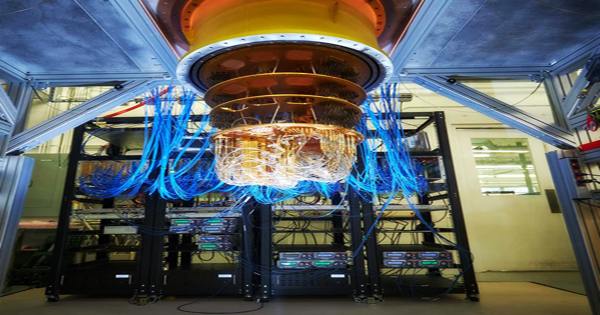
Finally, QAOA is intended to aid us in our quest for the fabled “quantum speedup,” the projected increase in processing speed that can be achieved with quantum computers rather than traditional ones. QAOA has a number of supporters, including Google, who have their sights set on quantum technologies and computing in the near future: in 2019, they developed Sycamore, a 53-qubit quantum processor, and used it to complete a task that a state-of-the-art classical supercomputer would take 10,000 years to complete. The identical process took Sycamore 200 seconds to complete.
Quantum computers are highly sensitive: heat, electromagnetic fields, and collisions with air molecules can cause a qubit to lose its quantum properties. This process, known as quantum decoherence, causes the system to crash, and it happens more quickly the more particles that are involved.
“But the barrier of ‘quantum speedup’ is all but rigid and it is being continuously reshaped by new research, also thanks to the progress in the development of more efficient classical algorithms,” says Carleo.
Carleo and Medvidović address a fundamental unresolved topic in the field: can algorithms operating on present and near-term quantum computers give a meaningful advantage over conventional algorithms for practical tasks? “If we are to answer that question, we first need to understand the limits of classical computing in simulating quantum systems,” says Carleo. This is particularly significant since the present generation of quantum processors makes mistakes when executing quantum “software,” limiting their ability to perform algorithms of restricted complexity.
The two researchers created a method that can roughly replicate the behavior of a particular class of algorithms known as variational quantum algorithms, which are means of determining the lowest energy state, or “ground state,” of a quantum system, using ordinary computers. QAOA is one of many quantum algorithms in this family, which experts believe are among the most promising prospects for “quantum advantage” in near-term quantum computers.
Qubits in quantum computers must be protected from extraneous influence by being physically isolated, kept cold, or zapped with precisely regulated energy pulses. To compensate for mistakes that sneak into the system, more qubits are required.
The method is based on the notion that contemporary machine-learning techniques, such as those used to learn complicated games like Go, may also be used to learn and replicate the inner workings of a quantum computer. Neural Network Quantum States, an artificial neural network created by Carleo and Matthias Troyer in 2016 and utilized for the first time to simulate QAOA, is the main instrument for these simulations. The findings are regarded to be in the realm of quantum computing, and they set a new bar for future quantum hardware development.
“Our work shows that the QAOA you can run on current and near-term quantum computers can be simulated, with good accuracy, on a classical computer too,” says Carleo. “However, this does not rule out the possibility of classically emulating all relevant quantum algorithms that can be performed on near-term quantum processors. Indeed, we expect that our method will be used to develop novel quantum algorithms that are both beneficial and difficult to mimic on conventional computers.”