Machine learning can be used to forecast amine emissions by training a model on historical data of amine emissions and the factors that influence them. The model can then be used to make predictions about future emissions based on new input data, such as weather conditions, energy consumption, and production processes. Some popular machine learning algorithms that can be used for this purpose include linear regression, random forest, and support vector regression. It is important to note that the quality of the predictions will depend on the quality and availability of the data used to train the model.
A machine learning approach has been developed by scientists to accurately predict potentially harmful amine emissions from carbon-capture-and-storage plants. Global warming is caused in part by the massive amount of carbon dioxide that we emit, primarily from power generation and industrial processes such as steel and cement production. Chemical engineers have been researching carbon capture, a process that can separate carbon dioxide and store it in ways that keep it out of the atmosphere, for quite some time.
This is done in dedicated carbon-capture plants, which use amines in their chemical process to capture carbon dioxide from natural gas processing and refining plants. Amines are also found in medicines, epoxy resins, and dyes.
We devised an experimental campaign to determine how and when amine emissions would occur. However, some of our experiments required the plant’s operators to intervene to ensure the plant was operating safely.
Professor Berend Smit
The issue is that amines may be harmful to the environment as well as a health hazard, so it is critical to reduce their impact. This necessitates accurate monitoring and prediction of amine emissions from a plant, which has proven difficult due to the complexity and variability of carbon-capture plants.
Using experimental data from a stress test at an actual plant in Germany, a team of scientists developed a machine-learning solution for forecasting amine emissions from carbon-capture plants. The research was led by Professor Berend Smit of EPFL’s School of Basic Sciences and Professor Susana Garcia of Heriot-Watt University’s Research Centre for Carbon Solutions in Scotland.
“The experiments were done in Niederhau?en, on one of the largest coal-fired power plants in Germany,” says Berend Smit. “And from this power plant, a slipstream is sent into a carbon capture pilot plant, where the next generation of amine solution has been tested for over a year. But one of the outstanding issues is that amines can be emitted with flue gas, and these amine emissions need to be controlled.”
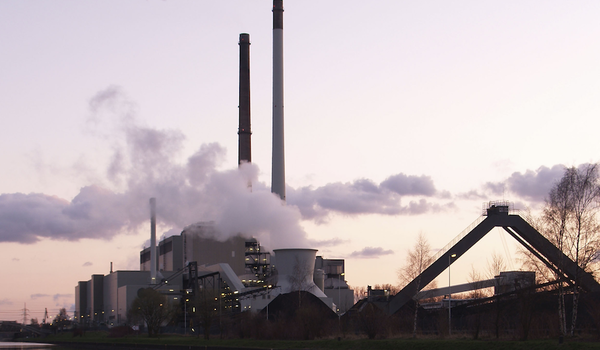
Professor Susana Garcia developed a stress test to study amine emissions under various process conditions in collaboration with the plant’s owner, RWE, and TNO in the Netherlands. Professor Garcia describes the test as follows: “We devised an experimental campaign to determine how and when amine emissions would occur. However, some of our experiments required the plant’s operators to intervene to ensure the plant was operating safely.”
These interventions led to the question of how to interpret the data. Are the amine emissions the result of the stress test itself, or have the interventions of the operators indirectly affected the emissions? This was further complicated by our general lack of understanding of the mechanisms behind amine emissions. “In short, we had an expensive and successful campaign that showed that amine emissions can be a problem, but no tools to further analyze the data,” says Smit.
He goes on to say: “When Susana Garcia told me about it, it sounded like an impossible problem to solve. She did, however, mention that they measured everything every five minutes and collected a lot of data. Kevin is the only person in my group who can solve impossible problems with data.” A PhD student, Kevin Maik Jablonka, devised a machine learning approach that transformed the amine emissions puzzle into a pattern-recognition problem.
“We wanted to know what the emissions would be if we did not have the stress test but only the operators’ interventions,” explains Smit. This is a similar issue as we can have in finance; for example, if you want to evaluate the effect of changes in the tax code, you would like to disentangle the effect of the tax code from, say, interventions caused by the crisis in Ukraine.”
Jablonka then used powerful machine learning to forecast future amine emissions based on data from the plant. He claims: “We were able to predict the emissions caused by the operators’ interventions and then separate them from those caused by the stress test using this model. Furthermore, we could use the model to run various scenarios for lowering these emissions.”
“Surprising” was the word used to describe the conclusion. The pilot plant, it turned out, was designed for pure amine, but the measuring experiments were conducted on a mixture of two amines: 2-amino-2-methyl-1-propanol and piperazine (CESAR1). The researchers discovered that the two amines react in opposite ways: reducing one increases the emissions of the other.