Pipeline elbow erosion detection is critical for maintaining pipeline integrity and preventing potential failures. While traditional methods such as visual inspection, ultrasonic testing, and magnetic particle inspection are widely used, new percussion methods can provide additional insights. A University of Houston engineering research team is developing a new percussion-based method for detecting pipeline elbow erosion. It is not a minor issue.
A veritable superhighway of piping beneath the earth’s surface transports corrosive liquids and elements such as carbon dioxide, hydrogen, methanol, and others for a variety of industrial needs ranging from oil well cementing to chemical mining. At each bend, a pipeline elbow is installed to guide the flow in new directions.
According to research, the mass loss of the pipeline elbow due to erosion is approximately 50 times greater than that of the straight pipe, and the wall thickness of the pipeline elbow becomes thinner with continuous operation. This could result in the pipeline elbow bursting or piercing, resulting in economic losses, pollution, and other safety issues.
The new method removes the need for the constant-contact sensor and professional operator and shows great applicability in different pipeline elbows with the same structure and dimension and is easy-to-implement, low-cost, and free of the installation of a constant-contact-sensor.
Jian Chen
Because 90-degree pipeline elbows are prone to erosion caused by the transported medium, detection of pipeline elbow erosion is critical to the pipeline system’s health. Most pipeline elbow erosion detection methods currently require the installation of a constant-contact sensor, which is limited in certain environments.
“We propose a novel detection method for pipeline elbow erosion, combining percussion, variational mode decomposition (VMD) and deep learning,” reports Gangbing Song, Moores Professor of Mechanical Engineering, in the journal Mechanical Systems and Signal Processing. “The new method removes the need for the constant-contact sensor and professional operator and shows great applicability in different pipeline elbows with the same structure and dimension and is easy-to-implement, low-cost, and free of the installation of a constant-contact-sensor.” Jian Chen, a doctoral student, and Lan Cao, a graduated master’s student, are the co-authors of the paper.
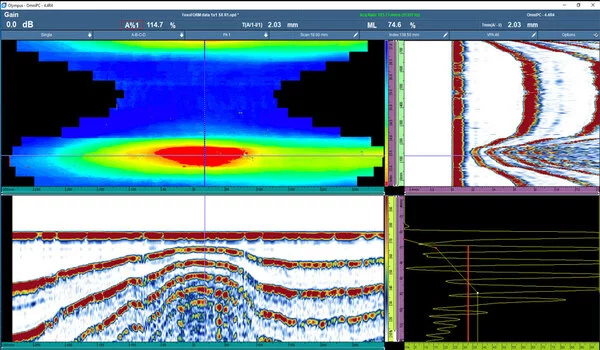
The method works by analyzing a sound produced by hitting a surface with VMD. VMD also divides the sound into seven distinct components or modes. The modes are then subjected to a machine learning technique known as multi-rocket. Multi-rocket essentially assists in the identification and selection of the most significant or representative component from the original sound produced by a single hit. The process’s goal is to improve the understanding and interpretation of the audio signal.
The team conducted two case studies on three pipeline elbows with similar structure and dimensions to validate the effectiveness of the proposed method.
All of the methods performed admirably in the first case study, with an accuracy of around 100% across six erosion levels. In the second case study, however, the proposed method demonstrated exceptional effectiveness, achieving accuracy greater than 90%. This outperformed other methods, both shallow and deep learning, with accuracies of less than 80%. In the second case study, the proposed method outperformed the others in terms of accurately classifying data.
The article is the first to investigate pipeline elbow erosion using the percussion method, machine learning, and deep learning. Song and his colleagues have applied for a patent on their invention, titled “Detecting Elbow Erosion by Percussion Method with Machine Learning.”