The use of very-large-scale integration (VLSI) systems containing electronic analog circuits to imitate neuro-biological architectures present in the nervous system is known as neuromorphic engineering. A neuromorphic computer/chip is any device that does calculations using physical artificial neurons (made of silicon). The word neuromorphic has recently been used to characterize analog, digital, mixed-mode analog/digital VLSI, and software systems that implement neural system models (for perception, motor control, or multisensory integration).
Researchers have disclosed a nano-sized neuromorphic memory device that emulates neurons and synapses in a unit cell at the same time, which is another step toward the objective of neuromorphic computing, which is designed to closely replicate the human brain with semiconductor devices.
Scientists have long been amazed by the human brain’s ability to comprehend enormous volumes of information while expending minimum energy. When there is a demand, the brain increases computation, but it quickly returns to a baseline level. Such efficiencies have never been conceivable in the world of silicon-based computing. Massive amounts of electrical energy are required to process big amounts of data. Furthermore, when AI and its brethren deep learning and machine learning enter the picture, the problem worsens exponentially.
Neurons and synapses interact with each other to establish cognitive functions such as memory and learning, so simulating both is an essential element for brain-inspired artificial intelligence.
Professor Keon Jae Lee
The goal of neuromorphic computing is to create artificial intelligence (AI) by emulating the mechanics of neurons and synapses seen in the human brain. Neuromorphic devices have been intensively researched because they are inspired by cognitive functions of the human brain that existing computers cannot deliver. However, present CMOS-based neuromorphic circuits simply connect artificial neurons and synapses without any synergistic interactions, and the simultaneous implementation of neurons and synapses remains a difficulty.
To address these issues, a research team led by Professor Keon Jae Lee from the Department of Materials Science and Engineering implemented the biological working mechanisms of humans by introducing the neuron-synapse interactions in a single memory cell, rather than the conventional approach of electrically connecting artificial neuronal and synaptic devices.
Similar to commercial graphics cards, the previously researched artificial synaptic devices were frequently used to accelerate parallel computations, demonstrating substantial variations from the operating systems of the human brain. The synergistic connections between neurons and synapses were incorporated in the neuromorphic memory device, simulating the mechanisms of the organic neural network. Furthermore, the new neuromorphic device can replace complex CMOS neuron circuits with a single device, resulting in increased scalability and cost-efficiency.
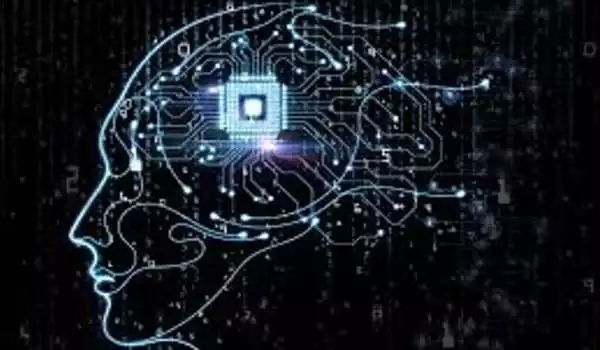
A complicated network of 100 billion neurons and 100 trillion synapses makes up the human brain. The functions and architecture of neurons and synapses can alter in response to external inputs, allowing them to adapt to their surroundings. The researchers created a neuromorphic system in which short-term and long-term memories coexist utilizing volatile and non-volatile memory devices that match the properties of neurons and synapses, respectively.
A threshold switch device serves as volatile memory, whereas phase-change memory serves as non-volatile memory. Two thin-film devices are combined without the use of intermediate electrodes to simulate the functional flexibility of neurons and synapses in neuromorphic memory.
The human brain is a fascinating evolutionary product. It has a low energy footprint of roughly 20 watts and can do complex tasks in milliseconds. While today’s CPUs and GPUs may far exceed the human brain in serial processing tasks, the process of transporting data from memory to a processor and back causes not only latency but also consumes massive amounts of energy. An average desktop computer consumes about 200 watts, however, some supercomputers consume up to 20 megawatts.
“Neurons and synapses interact with each other to establish cognitive functions such as memory and learning, so simulating both is an essential element for brain-inspired artificial intelligence,” said Professor Keon Jae Lee. “The developed neuromorphic memory device also mimics the retraining effect that allows quick learning of the forgotten information by implementing a positive feedback effect between neurons and synapses.”