A new study led by a researcher at the University of Buffalo has discovered how specific communication between different brain regions, known as brain connectivity, can serve as a biomarker for attention deficit hyperactivity disorder (ADHD). The study used a deep architecture with machine-learning classifiers to identify adults who had been diagnosed with ADHD as children many years before with 99 percent accuracy.
“This suggests that brain connectivity is a stable biomarker for ADHD, at least into childhood, even when an individual’s behavior has become more typical, possibly by adopting different strategies that obscure the underlying disorder,” said Chris McNorgan, the study’s lead author and an assistant professor of psychology in the UB College of Arts and Sciences.
The findings, published in the journal Frontiers in Physiology, have implications not only for detecting ADHD, a common but difficult-to-diagnose disorder but also for helping clinicians target treatments by understanding where patients fall on a broad-ranging continuum.
“Because certain pharmaceuticals react with specific pathways, understanding the different types of ADHD can help inform decisions about one medication versus others,” McNorgan, a neuroimaging and computational modeling expert, explained.
A patient may exhibit behavioral symptoms consistent with ADHD one day, but days later, those symptoms may not be present, or may not be present to the same degree. It could be the difference between having a good day and having a bad day. However, the ADHD brain connectivity signature appears to be more stable. The diagnostic flip-flop is not visible to us.
Chris McNorgan
Attention deficit disorder is the most common psychological disorder among school-aged children, but it is difficult to diagnose. Furthermore, the clinical definition of ADHD is complicated by the presence of multiple subtypes. When a patient returns for a follow-up evaluation, his or her clinical diagnosis of ADHD may change.
“A patient may exhibit behavioral symptoms consistent with ADHD one day, but days later, those symptoms may not be present, or may not be present to the same degree,” McNorgan explained. “It could be the difference between having a good day and having a bad day.” However, the ADHD brain connectivity signature appears to be more stable. The diagnostic flip-flop is not visible to us.”
Attention deficit disorder is the most common psychological disorder among school-aged children, but it is difficult to diagnose. Furthermore, the clinical definition of ADHD is complicated by the presence of multiple subtypes. When a patient returns for a follow-up evaluation, his or her clinical diagnosis of ADHD may change.
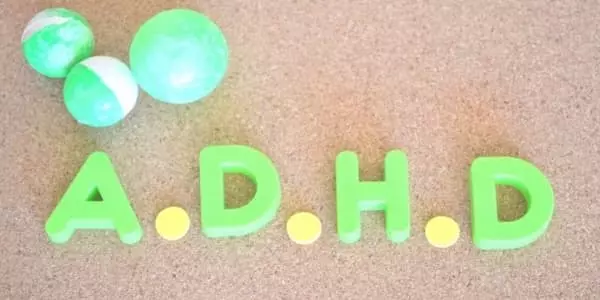
“A patient may exhibit behavioral symptoms consistent with ADHD one day, but days later, those symptoms may not be present, or may not be present to the same degree,” McNorgan explained. “It could be the difference between having a good day and having a bad day.” However, the ADHD brain connectivity signature appears to be more stable. The diagnostic flip-flop is not visible to us.”
“It’s by far the highest accuracy rate I’ve seen reported anywhere — it’s leagues ahead of anything that has come before it, and far ahead of anything that has been achieved with a behavioral assessment,” McNorgan said. “A number of factors are likely to have contributed to our superior classification performance.”
Previous studies that suggested a link between brain connectivity and ADHD relied on direct linear classification. This study looks at the relationships between something and what it predicts, such as coffee and performance.
Direct linear classification is effective for many ranges, but the relationship between coffee and performance, like behavioral symptoms and ADHD, is not linear. One or two cups of coffee may boost performance temporarily, but caffeine can have a negative impact on performance over time. According to McNorgan, nonlinear relationships exist when there is “too little or too much of a good thing.”
Deep learning networks are well-suited for detecting nonlinear conditional relationships. In the current study, ADHD was predicted based on communication patterns between groups of brain areas, such as A, B, and C. If regions A and B were highly connected, this could be predictive of ADHD; however, if these regions were also highly connected to region C, this would not be the case. These sorts of relationships are problematic for the most commonly used techniques, but not for deep-learning classifiers.
McNorgan’s model goes a step further by distinguishing between individuals with ADHD who perform normally or abnormally on the Iowa Gambling Task (IGT). The IGT is a behavioral paradigm that is similar to a casino card game in that it offers both high-risk and low-risk options and is commonly used to study and diagnose ADHD.
Traditional classification techniques can only make one classification at a time. McNorgan’s method elegantly connects ADHD diagnosis and IGT performance to provide a potential bridge that explains why both are related to brain wiring. Furthermore, while people with ADHD tend to make more risky choices in the IGT, this is not a universal determinant. Some people who do not have ADHD make riskier decisions than others.
“By differentiating both of these dimensions, this approach provides a mechanism for sub-classifying people with ADHD in ways that can allow for targeted treatments,” McNorgan said. “We can see where people fall on the spectrum.” Because different brain networks are implicated in people at either end of the spectrum, he says, this method opens the door to developing therapies that target specific brain networks.