Machine learning has been important in identifying sources of heterogeneity among cells in our bodies. The human body is made up of trillions of cells, and even within a single tissue or organ, individual cells can vary significantly. Understanding this diversity is critical for expanding our understanding of health, disease, and biological processes.
A team of South Korean scientists, lead by Professor KIM Jae Kyoung of the Biomedical Mathematics Group at the Institute for Basic Science (IBS-BIMAG), revealed the secrets of cell variability in our bodies. The findings of this study are predicted to have far-reaching implications, such as increasing the efficacy of chemotherapy treatments or establishing a new paradigm in the study of antibiotic-resistant microorganisms.
Our cells have a signaling system that responds to a variety of environmental stimuli, including antibiotics and variations in osmotic pressure. This signaling system is crucial for cell survival since it interacts with the external environment. Even cells with identical genetic information can respond differently to the same external stimuli, a phenomenon known as cellular heterogeneity.
The findings of this study are predicted to have far-reaching implications, such as increasing the efficacy of chemotherapy treatments or establishing a new paradigm in the study of antibiotic-resistant microorganisms.
KIM Jae Kyoung
Cellular heterogeneity is a popular research topic in medicine since it is known to impede the complete eradication of cancer cells by chemotherapeutic agents such as anticancer medicines. The sources of such variability and their relationship to the signaling system have remained a difficulty, as intermediate signaling system activities are unable to completely monitored with present experimental equipment.
The application of machine learning to study cellular heterogeneity has significantly accelerated our ability to unravel the complexity of biological systems. As technology continues to advance, these approaches will likely play an even more substantial role in furthering our understanding of cellular diversity and its implications for health and disease.
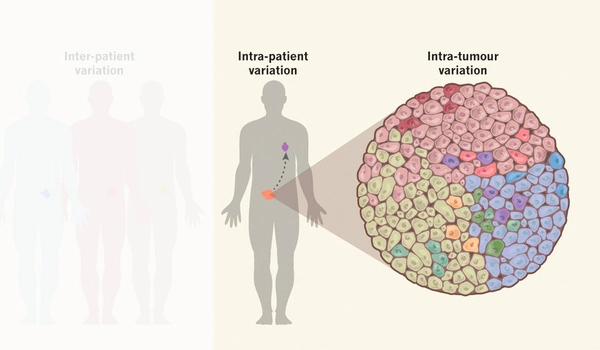
Professor Kim’s research team created a machine learning technology that employs artificial neural network topologies known as Density Physics-informed neural networks (Density-PINNs) to identify the sources of this heterogeneity. Density-PINNs use observable time-series data from cell responses to external stimuli to inversely estimate signaling system information. By applying Density-PINNs to actual experimental data of antibiotic responses in bacterial cells (Escherichia coli), the study team discovered that a parallel signaling system can diminish cell heterogeneity.
Professor Kim believes that this mathematical modeling and machine learning research will facilitate the enhancement of the understanding of cellular heterogeneity, which is crucial in cancer treatment. He expressed his hope that this achievement would lead to the development of improved cancer treatment strategies.
Dr. JO Hyeontae and Dr. HONG Hyukpyo participated as co-first authors in this research, which was published in the international journal Patterns (Impact Factor 6.5), a sister journal of Cell. The title of the paper is “Density Physics-informed Neural Networks Reveal Sources of Cell Heterogeneity in Signal Transduction.”