Geologists and researchers have been investigating the use of artificial intelligence to predict landslides. Landslides are geological hazards that can cause significant damage to property and loss of life. Traditional methods of landslide prediction typically rely on manual observation and analysis of geological and environmental factors, which can be time-consuming and not always accurate. AI can help improve the accuracy and efficiency of landslide prediction by analyzing massive amounts of data and identifying patterns that humans may not be able to detect.
Many factors influence where a landslide will occur, including the shape of the terrain, its slope and drainage areas, the material properties of soil and bedrock, and environmental conditions such as climate, rainfall, hydrology, and ground motion caused by earthquakes.
A new technique developed by geologists that uses artificial intelligence to better predict where and why landslides may occur could help to protect lives and property in some of the world’s most disaster-prone areas. The new method improves the accuracy and interpretability of AI-based machine-learning techniques while requiring far less computing power and being more widely applicable than traditional predictive models.
A new technique developed by UCLA geologists that uses artificial intelligence to better predict where and why landslides might occur could help protect lives and property in some of the world’s most disaster-prone areas.
The new method, described in a paper published in the journal Communications Earth & Environment, improves the accuracy and interpretability of AI-based machine-learning techniques, requires far less computing power, and is more broadly applicable than traditional predictive models.
DNNs will deliver a percentage likelihood of a landslide that may be accurate, but we are unable to figure out why and which specific variables were most important in causing the landslide.
Kevin Shao
The approach would be particularly valuable in places like California, the researchers say, where drought, wildfires and earthquakes create the perfect recipe for landslide disasters and where the situation is to expected to get worse as climate change brings stronger and wetter storms.
Many factors influence where a landslide will occur, including terrain shape, slope and drainage areas, soil and bedrock material properties, and environmental conditions such as climate, rainfall, hydrology, and ground motion caused by earthquakes. With so many variables to consider, predicting when and where a chunk of earth will lose its grip is as much an art as it is a science.
Traditionally, geologists have estimated a region’s landslide risk by incorporating these factors into physical and statistical models. With enough data, such models can make reasonably accurate predictions, but physical models are time and resource-intensive and cannot be applied across large areas, whereas statistical models provide little insight into how they evaluate various risk factors to make their predictions.
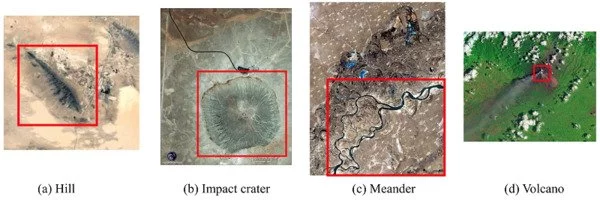
Using artificial intelligence to predict landslides
Deep neural networks, or DNNs, are AI machine-learning models that have been trained to predict landslides in recent years. When fed reams of landslide-related variables and historical landslide information, these large, interconnected networks of algorithms can process and “learn” from this data in record time, allowing them to make highly accurate predictions.
Nonetheless, despite their advantages in processing time and learning power, DNNs, like statistical models, do not “show their work,” making it difficult for researchers to interpret their predictions and identify which causative factors to target in order to prevent future landslides.
“DNNs will deliver a percentage likelihood of a landslide that may be accurate, but we are unable to figure out why and which specific variables were most important in causing the landslide,” said Kevin Shao, a doctoral student in Earth, planetary and space sciences and co-first author of the journal paper.
The problem, said co-first author Khalid Youssef, a former student of biomedical engineering and postdoctoral researcher at UCLA, is that the various network layers of DNNs constantly feed into one another during the learning process, and untangling their analysis is impossible. The UCLA researchers’ new method aimed to address that.
“We sought to enable a clear separation of the results from the different data inputs, which would make the analysis far more useful in determining which factors are the most important contributors to natural disasters,” he said.
Youssef and Shao collaborated with co-corresponding authors Seulgi Moon, an associate professor of Earth, planetary, and space sciences at UCLA, and Louis Bouchard, a UCLA professor of chemistry and bioengineering, to develop a method for decoupling the analytic power of DNNs from their complex adaptive nature in order to deliver more actionable results.
Their method employs a type of AI known as a superposable neural network, or SNN, in which the network’s different layers run concurrently, retaining the ability to assess the complex relationships between data inputs and output results but only converging at the very end to yield the prediction.
The researchers fed 15 geospatial and climatic variables relevant to the eastern Himalaya mountains into the SNN. The region was chosen because the majority of human losses due to landslides occur in Asia, with the Himalayas accounting for a sizable portion. The SNN model was able to predict landslide susceptibility in Himalayan areas with accuracy comparable to DNNs, but most importantly, the researchers were able to tease apart the variables to see which ones played a larger role in producing the results.
“Identifying the exact trigger for a landslide will always require field measurements and historical records of soil, hydrologic, and climate conditions, such as rainfall amount and intensity, which can be difficult to obtain in remote places like the Himalayas,” Moon said. “Nonetheless, our new AI prediction model can identify key variables and quantify their contributions to landslide susceptibility.”
The new AI program developed by the researchers also requires far fewer computer resources than DNNs and can run effectively with relatively little computing power.