Machine learning can be a powerful tool to help find reliable and low-cost solar cells. One approach could be to use machine learning algorithms to analyze large amounts of data on different types of solar cells, including their materials, manufacturing processes, and performance characteristics.
Hybrid perovskites are organic-inorganic molecules that have gotten a lot of attention in the last decade because of their potential use in renewable energy. Some are as efficient as silicon in the production of solar cells, but they are less expensive and lighter, potentially allowing a wide range of applications, including light-emitting devices. When exposed to moisture, oxygen, light, heat, and voltage, they degrade much faster than silicon. Researchers used machine learning and high-throughput experiments to select perovskites with the best properties from a large field of possible structures.
Machine learning is being used by researchers at the University of California, Davis College of Engineering to identify new materials for high-efficiency solar cells. Using high-throughput experiments and machine learning-based algorithms, they discovered that it is possible to forecast the dynamic behavior of materials with high accuracy without performing as many experiments.
The research appears on the cover of the April issue of ACS Energy Letters.
These findings show that we can use machine learning to identify candidate materials and appropriate conditions to prevent degradation in perovskites. The experiments will then be expanded to quantify combinations of multiple environmental factors. Our paradigm is unique, and I’m looking forward to seeing the upcoming measurements.
Marina Leite
According to Marina Leite, associate professor of materials science and engineering at UC Davis and senior author on the paper, hybrid perovskites are organic-inorganic molecules that have received a lot of attention in the last 10 years for their potential use in renewable energy. Some are as efficient as silicon in the production of solar cells, but they are less expensive and lighter, potentially allowing a wide range of applications, including light-emitting devices.
The fact that perovskite devices degrade much faster than silicon when exposed to moisture, oxygen, light, heat, and voltage is a major challenge in the field. The challenge is determining which perovskites combine high-efficiency performance with environmental resilience.
Perovskites have a general structure of ABX3, where A is an organic (carbon-based) or inorganic group, B is lead or tin, and X is a halide (based on chlorine, iodine or fluorine or a combination). Therefore, “the number of possible chemical combinations alone is enormous,” Leite said. Further, they need to be assessed against multiple environmental conditions, alone and in combination, which results in a hyperparameter space that cannot be explored using conventional trial-and-error methods.
“The chemical parameter space is enormous,” Leite said. “To test them all would be very time consuming and tedious.”
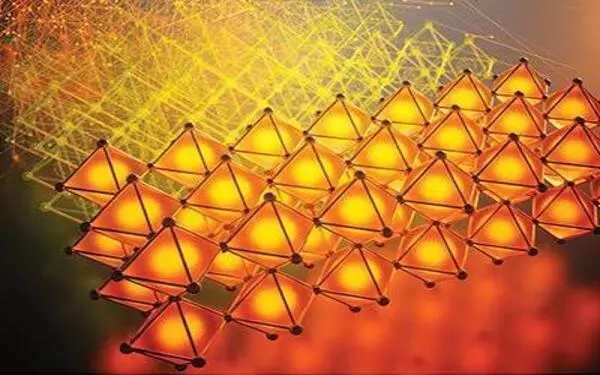
High throughput experiments and machine learning
Leite and graduate students Meghna Srivastava and Abigail Hering decide to test whether machine learning algorithms can be effective in testing and predicting the effects of moisture on material degradation as a first and critical step toward solving these challenges.
Srivastava and Hering developed an automated, high-throughput system to compare the photoluminescence efficiency of five different perovskite films to the conditions of Sacramento summer days. In a week, they were able to collect over 7,000 measurements, amassing enough data for a reliable training set.
They used this data to train three different machine learning algorithms: a linear regression model, a neural network and a statistical model called SARIMAX. They compared the predictions of the models to physical results measured in the lab. The SARIMAX model showed best performance with a 90 percent match to observed results during a window of 50-plus hours.
“These findings show that we can use machine learning to identify candidate materials and appropriate conditions to prevent degradation in perovskites,” Leite said. The experiments will then be expanded to quantify combinations of multiple environmental factors.
According to Leite, the perovskite film is only one component of a complete photovoltaic cell. The same machine learning method could be used to predict the behavior of an entire device.
“Our paradigm is unique, and I’m looking forward to seeing the upcoming measurements.” “I am also very proud of the students’ perseverance during the pandemic,” Leite said.