Neural-Fly technology has the potential to shape the future of package delivery drones and flying autos. Drones, or autonomous flying vehicles, will need to learn to navigate real-world weather and wind conditions in order to be genuinely useful.
Drones are now flown under controlled conditions, with no wind, or by people using remote controls. Drones have been taught to fly in formation in open air, however these flights are normally carried out under perfect settings and circumstances.
However, in order for drones to autonomously execute important but mundane duties like delivering parcels or airlifting injured drivers from a traffic collision, they must be able to adapt to wind conditions in real time – in meteorological terms, they must be able to roll with the punches.
To meet this problem, a team of Caltech engineers created Neural-Fly, a deep-learning technology that can assist drones in dealing with new and unfamiliar wind conditions in real time by just altering a few critical parameters.
A research published in Science Robotics describes Neural-Fly. Soon-Jo Chung, Bren Professor of Aerospace and Control and Dynamical Systems and Jet Propulsion Laboratory Research Scientist, is the corresponding author. The co-first authors are Caltech graduate students Michael O’Connell (MS ’18) and Guanya Shi.
Rather than attempting to qualify and quantify every effect of the turbulent and unpredictable wind conditions that we frequently encounter in air travel, we instead employ a combined approach of deep learning and adaptive control that allows the aircraft to learn from previous experiences and adapt to new conditions on the fly with stability and robustness guarantees.
Soon-Jo Chung
Neural-Fly was tested at Caltech’s Center for Autonomous Systems and Technologies’ (CAST) Real Weather Wind Tunnel, an unique 10-foot-by-10-foot array of more than 1,200 tiny computer-controlled fans that allows engineers to replicate everything from a moderate gust to a gale.
“The problem is that the direct and unique influence of different wind conditions on aircraft dynamics, performance, and stability cannot be effectively captured by a simple mathematical model,” Chung explains. “Rather than attempting to qualify and quantify every effect of the turbulent and unpredictable wind conditions that we frequently encounter in air travel, we instead employ a combined approach of deep learning and adaptive control that allows the aircraft to learn from previous experiences and adapt to new conditions on the fly with stability and robustness guarantees.”
O’Connell continues: “We have many different fluid mechanics models, but attaining the correct model fidelity and tailoring that model for each vehicle, wind situation, and operating mode is difficult. Existing machine learning algorithms, on the other hand, require massive quantities of data to train and do not match state-of-the-art flight performance produced using traditional physics-based methods. Furthermore, real-time adaptation of a complete deep neural network is a massive, if not currently impossible, undertaking.”
According to the researchers, Neural-Fly overcomes these issues by employing a so-called separation technique, in which only a few parameters of the neural network must be changed in real time.
“We achieve this with our unique meta-learning technique, which pre-trains the neural network so that only these critical parameters need to be updated to successfully capture the changing environment,” Shi explains.
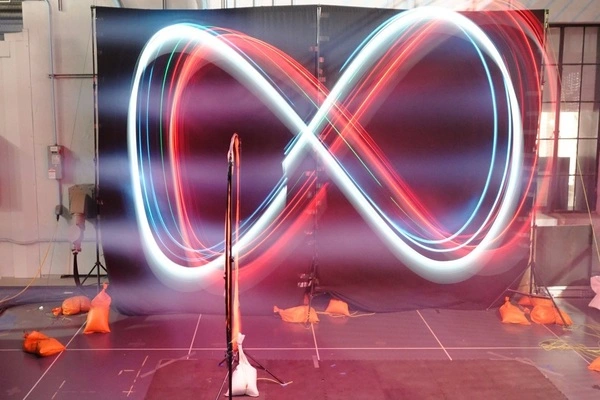
After only 12 minutes of flight time, autonomous quadrotor drones outfitted with Neural-Fly learn how to respond to high winds so successfully that their performance improves dramatically (as measured by their ability to precisely follow a flight path). The error rate following that flying path is approximately 2.5 to 4 times lower when compared to current state-of-the-art drones equipped with similar adaptive control algorithms that detect and respond to aerodynamic forces but lack deep neural networks.
Neural-Fly is built on prior systems known as Neural-Lander and Neural-Swarm, which were created in partnership with Caltech’s Yisong Yue, Professor of Computing and Mathematical Sciences, and Anima Anandkumar, Bren Professor of Computing and Mathematical Sciences. Neural-Lander also used a deep-learning method to track the drone’s position and speed as it landed, modifying its landing trajectory and rotor speed to compensate for the rotors’ backwash from the ground and achieve the smoothest possible landing; Neural-Swarm taught drones to fly autonomously in close proximity to one another.
Though landing appears to be more difficult than flying, Neural-Fly, unlike previous systems, can learn in real time. As a result, it can adjust to changes in wind on the fly and does not require post-processing. Neural-Fly performed just as well in flight tests outside of the CAST facility as it did in the wind tunnel. Furthermore, the team demonstrated that flight data collected by one drone can be transmitted to another, so creating a knowledge pool for autonomous cars.
Test drones were tasked with flying in a pre-defined figure-eight pattern while being blasted with winds up to 12.1 meters per second — nearly 27 miles per hour, or a six on the Beaufort scale of wind speeds. This is classified as a “strong breeze,” making it difficult to utilize an umbrella. It is barely below a “moderate gale,” in which it would be impossible to move and entire trees would sway. This wind speed is twice as fast as the speeds seen by the drone during neural network training, implying that Neural-Fly might extrapolate and generalize well to unexpected and harsher conditions.
The drones were outfitted with a typical, off-the-shelf flight control computer utilized by the drone research and enthusiast communities. Neural-Fly was built into an onboard Raspberry Pi 4 computer, which is the size of a credit card and costs roughly $20.