Researchers from ETH Zurich, the University of Zurich, and Empa have created a novel material for an electronic component that is more versatile than its predecessors. Such components will aid in the development of electronic circuits that mimic the human brain and are more efficient at completing machine-learning tasks.
The human brain is extremely energy efficient when compared to computers. Scientists are now gaining inspiration for innovative computing systems from how the brain and its linked neurons function. They anticipate that these brain-inspired computing systems will be more energy efficient than traditional ones and will perform better at machine-learning tasks.
Scientists hope to integrate storage and processing in a single electrical component type known as a memristor, similar to how neurons in the brain are responsible for both data storage and data processing. Their expectation is that this will aid in achieving improved efficiency, as transferring data between the CPU and storage, as traditional computers do, is the primary source of high energy consumption in machine learning applications.
Researchers from ETH Zurich, the University of Zurich, and Empa have now developed a novel design for a memristor that may be utilized in a much broader range of applications than existing memristors. “There are various operation modes for memristors, and it is advantageous to be able to exploit all of these modes depending on the architecture of an artificial neural network,” ETH postdoc Rohit John explains. “However, earlier traditional memristors had to be programmed for one of these modes in advance.” The new memristors developed by Zurich researchers may now effortlessly switch between two operation modes while in use: one in which the signal weakens over time and dies (volatile mode), and one in which the signal remains constant (non-volatile mode).
There are various operation modes for memristors, and it is advantageous to be able to exploit all of these modes depending on the architecture of an artificial neural network. However, earlier traditional memristors had to be programmed for one of these modes in advance.
Rohit John
Just like in the brain
“These two operation modes can also be observed in the human brain,” says John. On the one hand, biological neurotransmitters are used to convey synaptic inputs from neuron to neuron. These stimuli begin strongly and gradually weaken. New synaptic connections to other neurons, on the other hand, emerge in the brain as we learn. These bonds last longer.
In 2020, John, a postdoctoral researcher in the group of ETH Professor Maksym Kovalenko, was awarded an ETH fellowship for excellent postdoctoral researchers. John did this study alongside Yiit Demira, a doctorate student in Professor Giacomo Indiveri’s department at the University of Zurich and ETH Zurich’s Institute for Neuroinformatics.
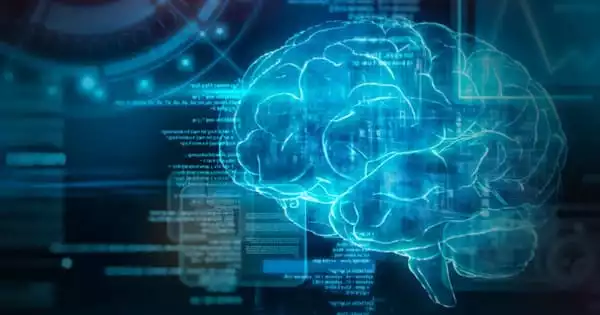
Semiconductor known from solar cells
The memristors produced by the researchers are made of halide perovskite nanocrystals, a semiconductor material well known for its usage in solar cells. “In these novel memristors, ‘nerve conduction’ is mediated by momentarily or permanently stringing together silver ions from an electrode to produce a nanofilament penetrating the perovskite structure through which current can flow,” Kovalenko adds.
This process can be controlled to make the silver-ion filament thin, allowing it to gradually degrade back into individual silver ions (volatile mode), or thick and permanent (non-volatile mode). The intensity of the current applied to the memristor controls this: a weak current activates the volatile mode, whereas a large current activates the non-volatile mode.
New toolkit for neuroinformaticians
“To the best of our knowledge, this is the first memristor that can be switched between volatile and non-volatile modes on demand,” Demira says. This indicates that in the future, computer chips with memristors that support both modes will be available. This is a significant advancement because it is typically not possible to mix multiple types of memristors on a single chip.
The researchers evaluated 25 of these novel memristors and took 20,000 measurements with them as part of their study, which was published in the journal Nature Communicationscall made. They were able to simulate a computational challenge on a complex network in this manner. The task included categorizing a variety of distinct neuron spikes as one of four predefined patterns.
These memristors will need to be further optimized before they can be employed in computer technology. However, as Indiveri points out, such components are equally crucial for neuroinformatics research: “These components are more similar to genuine neurons than the preceding ones. As a result, they enable neuroinformatics researchers to better test ideas and, hopefully, get a better grasp of the computing principles of genuine neural circuits in people and animals.”