This article describe about Belief Propagation, which is commonly used in artificial intelligence and information theory and has demonstrated empirical success in numerous applications including low-density parity-check codes, turbo codes, free energy approximation, and satisfiability. It exist for several types of graphical models, like as Bayesian networks and Markov random fields, in particular. Belief Propagation algorithms are normally presented as message update equations on a factor graph, involving messages between variable nodes and their neighboring factor nodes and vice versa.
Belief Propagation
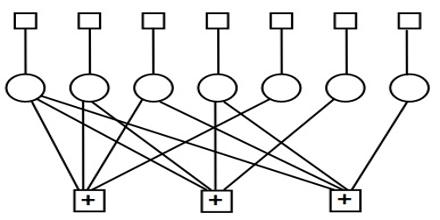