Researchers at Texas A&M University established a system to measure community recovery following natural disasters in near real-time by studying people’s visitation patterns to vital establishments such as pharmacies, religious centers, and grocery shops during Hurricane Harvey. They claimed that the information gained from their analysis will assist federal agencies in allocating resources evenly among disaster-affected communities.
“After a natural catastrophic catastrophe, neighboring towns can be impacted extremely differently,” said Dr. Ali Mostafavi, associate professor in the Zachry Department of Civil and Environmental Engineering and director of the Urban Resilience.AI Lab. “As a result, we need to identify which places will recover faster than others and which areas are more impacted than others so that we can direct more resources to those areas that require them the most.”
The researchers published their findings in Interface, a journal of The Royal Society.
The traditional metric for quantifying how communities recover from natural disasters is resilience, which is defined as a community’s ability to return to its pre-disaster status. As a result, elements such as the accessibility and distribution of resources, the connectivity between inhabitants within a community, and the level of community readiness for an unforeseen tragedy are crucial in measuring resilience.
Recovery information is actually needed in a speedier and more near-real-time approach for areas that are trailing in the recovery process for federal agencies providing cash. The solution, we reasoned, was to explore for developing data sources other than surveys that could provide more granular insights regarding community recovery at a scale that had not previously been investigated.
Dr. Ali Mostafavi
Surveys are the most common technique to collect the data needed to determine resilience. Among the issues considered are how and to what extent enterprises or homes were affected by the natural disaster, as well as the level of recovery. However, Mostafavi stated that, while these survey-based procedures are incredibly valuable, they take a long time to carry out, with the results of the survey becoming accessible months after the accident.
“Recovery information is actually needed in a speedier and more near-real-time approach for areas that are trailing in the recovery process for federal agencies providing cash,” said Mostafavi. “The solution, we reasoned, was to explore for developing data sources other than surveys that could provide more granular insights regarding community recovery at a scale that had not previously been investigated.”
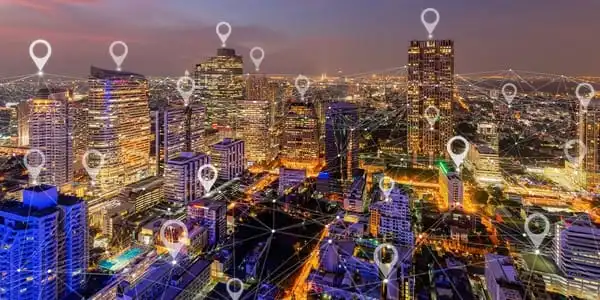
Mostafavi and his colleagues resorted to community-level big data, specifically information acquired by companies that track visits to areas inside a perimeter using anonymized mobile phone data. The researchers, in particular, collaborated with a business called SafeGraph to collect location data for people in Harris County, Texas, during Hurricane Harvey. As a first stage, they identified “points of interest,” which corresponded to the locations of establishments such as hospitals, gas stations, and stores that may see a change in visitor flow as a result of the hurricane.
The researchers then mined the large data to determine the amount of visitors to each area of attraction prior to and during the hurricane. They assessed the time required for visits to return to pre-disaster levels for various municipalities in Harris County, as well as the general resilience, which is the cumulative resilience of each place of interest-based on the % change in the number of visitors due to the hurricane.
According to their findings, communities with inadequate resilience endured higher flooding. However, their findings revealed that the level of impact did not always correlate with recovery.
“It’s natural to expect, for example, those firms harmed the most will recover more slowly, but this was not the case,” said Mostafavi. “There were certain areas where visitors dropped dramatically, but they quickly returned. Others, however, were less affected but took longer to recover, demonstrating the importance of both time and general resilience in assessing a community’s recovery.”
Another key discovery, according to the researchers, is that places close to those that have flooded are also impacted, implying that the spatial reach of flooding extends beyond flooded areas.
“While we focused on Hurricane Harvey for this study, our paradigm is relevant to any other natural disaster,” Mostafavi explained. “However, as a next step, we’d like to develop an intelligent dashboard that would indicate the rate of recovery and effects in various locations in near real-time, as well as predict the possibility of future access disruption and recovery patterns following a major downpour.”