Current neural network algorithms generate excellent outcomes that aid in the resolution of a vast array of issues. The electrical gadgets that operate these algorithms, on the other hand, still demand much too much computing power. When it comes to processing sensory information or interacting with the environment in real-time, these artificial intelligence (AI) systems simply cannot match with the human brain.
Artificial neural networks have a layered design, similar to the biological neural network in the human body, and each network node (connection point) may process input and send output to other nodes in the network. The hierarchical structure of neurons in organic sensory systems is represented in artificial neurons.
The neuromorphic chip detects high-frequency oscillations
Neuromorphic engineering is a promising new method for bridging the artificial-natural intelligence divide. This method was employed by an interdisciplinary research team from the University of Zurich, the ETH Zurich, and the University Hospital Zurich to build a chip based on neuromorphic technology that detects complicated biosignals reliably and precisely.
The scientists were able to effectively detect previously documented high-frequency oscillations (HFOs) using this approach. These unique waves have proved to be useful indicators for identifying the brain tissue that produces epileptic seizures, as evaluated by an intracranial electroencephalogram (iEEG).
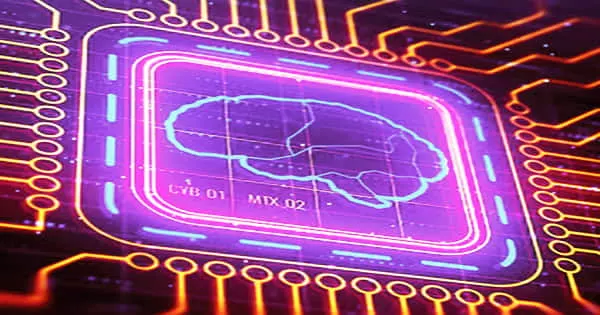
When neurons process input in both artificial and biological networks, they decide whether the output should be passed on to the next layer as input. The choice of whether or not to transmit information is known as bias, and it is governed by a system-built activation function.
Our design allows us to recognize spatiotemporal patterns in biological signals in real-time.
Giacomo Indiveri
Complex, compact and energy-efficient
The researchers created an algorithm that identifies HFOs by imitating the brain’s natural neural network: a miniature “spiking neural network” (SNN). The SNN was then implemented in a fingernail-sized piece of hardware that accepts neural impulses via electrodes and is tremendously energy efficient in comparison to traditional computers. This allows for calculations with extremely high temporal resolution without the need of the internet or cloud computing.
“Our design allows us to recognize spatiotemporal patterns in biological signals in real-time,” says Giacomo Indiveri, professor at the Institute for Neuroinformatics of UZH and ETH Zur-ich.
Measuring HFOs in operating theaters and outside of hospitals
The researchers now intend to apply these results to the development of an electronic system that can consistently detect and monitor HFOs in real-time. The device might improve the outcome of neurosurgical procedures when employed as an extra diagnostic tool in operating rooms.
However, this isn’t the only sector in which HFO recognition can be useful. The team’s long-term goal is to create an epilepsy monitoring system that can be used outside of the hospital and can evaluate signals from a large number of electrodes over a period of weeks or months.
“We want to integrate low-energy, wireless data communications in the design to connect it to a cellphone, for example,” says Indiveri. Johannes Sarnthein, a neurophysiologist at University Hospital Zurich, elaborates: “A portable or implantable chip such as this could identify periods with a higher or lower rate of incidence of seizures, which would enable us to deliver personalized medicine.”
The Zurich Center of Epileptology and Epilepsy Surgery is a collaboration between University Hospital Zurich, the Swiss Epilepsy Clinic, and the University Children’s Hospital Zurich that conducts epilepsy research.