A study team has created an optical computing system for AI and machine learning that not only mitigates the noise inherent in optical computing but also uses part of it as input to assist increase the creative output of the system’s artificial neural network.
Artificial intelligence and machine learning are currently influencing our lives in a variety of tiny but significant ways. AI and machine learning systems, for example, recommend content that we might enjoy through streaming services such as Netflix and Spotify.
These technologies are expected to have an even greater impact on society in the near future through activities such as driving fully driverless vehicles, enabling sophisticated scientific research, and facilitating medical discoveries.
However, the computers needed for AI and machine learning consume a significant amount of energy. The need for computer power connected to these technologies is currently increasing every three to four months. And cloud computing data centers, which are used by AI and machine learning applications all around the world, are already consuming more electricity per year than certain small countries. This amount of energy demand is clearly unsustainable.
We constructed an optical computer that is quicker than a typical digital computer. In addition, this optical computer can develop new things based on random inputs provided by the optical noise that most researchers attempted to avoid.
Lead Author Changming Wu
A team of researchers led by the University of Washington has created new optical computing hardware for AI and machine learning that is far faster and uses far less energy than conventional electronics. Another issue addressed in the study is the ‘noise’ inherent in optical computing, which can interfere with computation precision.
The team showcases an optical computing system for AI and machine learning in new research published in Science Advances that not only mitigates noise but also uses some of it as input to assist increase the creative output of the system’s artificial neural network.
“We constructed an optical computer that is quicker than a typical digital computer,” said lead author Changming Wu, a doctorate student in electrical and computer engineering at the University of Washington. “In addition, this optical computer can develop new things based on random inputs provided by the optical noise that most researchers attempted to avoid.”
Optical computing noise is primarily caused by stray light particles, or photons, generated by the functioning of lasers within the device as well as background thermal radiation. To target noise, the researchers linked their optical computing core to a Generative Adversarial Network, a form of machine learning network.
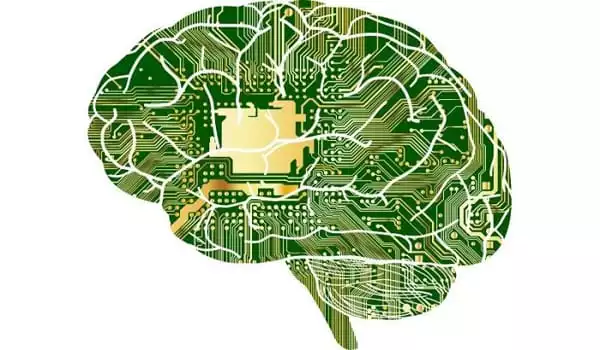
The team experimented with several noise reduction approaches, including using some of the noise generated by the optical computer core as random inputs for the GAN. The team, for example, handed the GAN the job of learning how to handwrite the number “7” in the manner of a human. The optical computer could not simply print the number in a predetermined font. It had to learn the task in the same way that a child would, by looking at visual samples of handwriting and practicing until it could accurately write the number. Of course the optical computer didn’t have a human hand for writing, so its form of “handwriting” was to generate digital images that had a style similar to the samples it had studied, but were not identical to them.
“Instead of teaching the network to read handwritten numbers, we trained it to learn to write numbers, emulating visual samples of handwriting that it was trained on,” said senior author Mo Li, an electrical and computer engineering professor at the University of Washington. “We also demonstrated, with the assistance of our computer science researchers at Duke University, that the GAN can offset the harmful impact of optical computing hardware sounds by employing a training technique that is resistant to mistakes and noises. Furthermore, the network uses the noises as the random input required to generate output instances.”
The GAN practiced writing “7” until it could do it successfully after learning from handwritten samples of the number seven from a normal AI-training picture collection. It developed its own particular writing style along the way and could write numbers from one to ten in computer simulations.
The device will next be built on a larger scale utilizing current semiconductor manufacturing methods. To attain wafer-scale technology, the team intends to use an industrial semiconductor foundry rather than build the next generation of the device in a lab. A larger-scale gadget will boost performance even more and enable the study team to undertake more complex activities beyond handwriting generation, such as making artwork and even videos.
“This optical system represents a computer hardware architecture that can enhance the creativity of artificial neural networks used in AI and machine learning,” Li explained. “More importantly, it demonstrates the viability of this system at a large scale where noise and errors can be mitigated and even harnessed. AI applications are expanding at such a rapid pace that their energy consumption will become unsustainable in the future. This technology has the potential to assist cut energy usage, making AI and machine learning more environmentally friendly – as well as very fast, reaching higher overall performance.”