An artificial intelligence model can now detect and predict the turbulent motions occurring within the Sun’s atmosphere. This neural network was created by a team of Japanese researchers and fed with vertical motion and temperature data collected from the surface of the solar photosphere. The solar photosphere is the Sun’s surface, as well as the lowest layer of the solar atmosphere.
Solar flares, coronal mass ejections, and sunspots all originate and occur on the solar photosphere. A close examination reveals that the solar photosphere is not uniform. The variable surface of the Sun is made up of granules, which are the top layers of convection cells in the solar plasma.
A newly developed neural network can accurately predict hidden turbulent motion in the Sun’s atmosphere. The AI model correctly identified turbulent horizontal motion below the surface when fed only temperature and vertical motion data collected from the surface of the solar photosphere. This could aid our understanding of solar convection and the processes that cause explosions and jets to erupt from the Sun.
We discovered that the rapid decrease in coherence spectrum occurred on scales lower than the energy injection scales, which were characterized by the peaks of the power spectra of the vertical velocities, by comparing the results of the three convection models. This implies that the network was not properly trained to reproduce the velocity fields generated by turbulent cascades at small scales.
A neural network deep learning technique was developed by scientists to extract hidden turbulent motion information from Sun observations. Tests on three different sets of simulation data revealed that horizontal motion can be inferred from temperature and vertical motion data. This technique will benefit solar astronomy and other fields such as plasma physics, fusion science, and fluid dynamics.
The Sun is critical to the Sustainable Development Goal of Affordable and Clean Energy, both as a source of solar power and as a natural example of fusion energy. The amount of data we can collect limits our understanding of the Sun. The temperature and vertical motion of solar plasma, a gas so hot that the component atoms break down into electrons and ions, are relatively easy to observe. However, determining the horizontal motion is difficult.
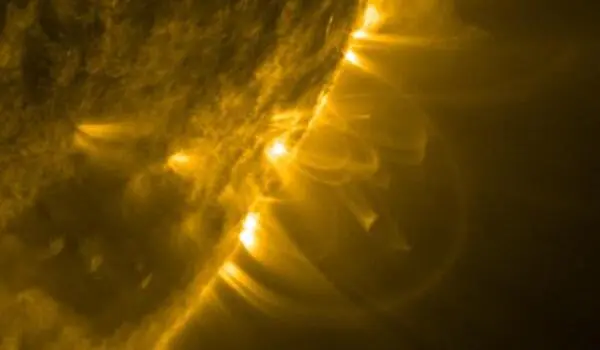
To address this issue, a team of scientists led by the National Astronomical Observatory of Japan and the National Institute for Fusion Science developed a neural network model and fed it data from three different simulations of plasma turbulence. After training, the neural network was able to correctly infer the horizontal motion given only the vertical motion and the temperature.
The team also developed a novel coherence spectrum to evaluate the performance of the output at different size scales. This new analysis showed that the method succeeded at predicting the large-scale patterns in the horizontal turbulent motion, but had trouble with small features. The team is now working to improve the performance a small scales. It is hoped that this method can be applied to future high-resolution solar observations, such as those expected from the SUNRISE-3 balloon telescope, as well as to laboratory plasmas, such as those created in fusion science research for new energy.
Ishikawa and colleagues created numerical simulations of plasma turbulence and trained their neural network using three different sets of simulation data. They discovered that using only temperature and vertical flow data, the AI was able to accurately describe horizontal flows in simulations that would be undetectable on the real Sun.
This means we could feed it solar data and expect the results to be consistent with what is actually happening on our fascinating, perilous star.
The neural network, on the other hand, requires some fine-tuning. While the AI was capable of detecting large-scale flows, it struggled to identify smaller features. Because the accuracy of small-scale turbulence is critical for some calculations, the researchers believe that resolving this issue should be the next step in developing their software.
“We discovered that the rapid decrease in coherence spectrum occurred on scales lower than the energy injection scales, which were characterized by the peaks of the power spectra of the vertical velocities, by comparing the results of the three convection models. This implies that the network was not properly trained to reproduce the velocity fields generated by turbulent cascades at small scales” They wrote something in their paper.