The researchers used chiral (twisted) magnets as their computational medium and discovered that the physical properties of these materials could be adapted to suit different machine-learning tasks by applying an external magnetic field and changing temperature.
A new study led by UCL and Imperial College London researchers has brought a type of brain-inspired computing that exploits the intrinsic physical properties of a material to dramatically reduce energy use one step closer to reality.
An international team of researchers used chiral (twisted) magnets as their computational medium in the new study, which was published in the journal Nature Materials, and discovered that by applying an external magnetic field and changing temperature, the physical properties of these materials could be adapted to suit different machine-learning tasks.
This approach, known as physical reservoir computing, has been limited in the past due to its lack of reconfigurability. This is because the physical properties of a material may allow it to excel at a subset of computing tasks but not others.
This work brings us a step closer to realizing the full potential of physical reservoirs to create computers that not only require significantly less energy but also adapt their computational properties to perform optimally across various tasks, just like our brains.
Dr Oscar Lee
An international team of researchers used chiral (twisted) magnets as their computational medium in the new study, which was published in the journal Nature Materials, and discovered that by applying an external magnetic field and changing temperature, the physical properties of these materials could be adapted to suit different machine-learning tasks.
“This work brings us a step closer to realizing the full potential of physical reservoirs to create computers that not only require significantly less energy but also adapt their computational properties to perform optimally across various tasks, just like our brains,” said Dr. Oscar Lee (London Centre for Nanotechnology at UCL and UCL Department of Electronic & Electrical Engineering).
“The next step is to identify materials and device architectures that are commercially viable and scalable.”
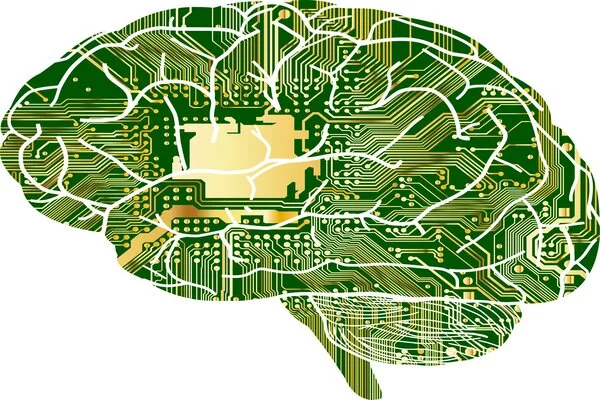
Traditional computing requires a lot of electricity. This is due in part to the fact that it has separate units for data storage and processing, requiring information to be constantly shuffled between the two, wasting energy and producing heat. This is especially problematic for machine learning, which requires large datasets to be processed. Training a large AI model can result in hundreds of tonnes of CO2.
Physical reservoir computing is one of several neuromorphic (or brain inspired) approaches aimed at eliminating the need for separate memory and processing units, allowing for more efficient data processing. Physical reservoir computing, in addition to being a more sustainable alternative to traditional computing, could be integrated into existing circuitry to provide additional capabilities that are also energy efficient.
The team used a vector network analyzer to determine the energy absorption of chiral magnets at different magnetic field strengths and temperatures ranging from -269 °C to room temperature in the study, which included researchers from Japan and Germany.
They discovered that different magnetic phases of chiral magnets excelled at various computing tasks. The skyrmion phase, in which magnetised particles swirl in a vortex-like pattern, possessed a powerful memory capacity suitable for forecasting tasks. Meanwhile, the conical phase had little memory, but its non-linearity was ideal for transformation tasks and classification, such as determining whether an animal is a cat or a dog.