Researchers can now forecast the activity of individual neurons without taking a single measurement in a living brain, thanks to maps of neural connections and artificial intelligence technologies.
For decades, neuroscientists have spent many hours in the lab methodically tracking the activity of neurons in living animals in order to figure out how the brain controls behavior. These investigations have provided ground-breaking insights into how the brain functions, but they have only scratched the surface, leaving much of the brain unknown.
Researchers are now utilizing artificial intelligence and the connectome, a map of neurons and their connections derived from brain tissue, to predict the role of neurons in the real brain. Researchers constructed an AI simulation of the fruit fly visual system that can predict the activity of every neuron in the circuit using just information about the connections of a neural circuit obtained from the fruit fly visual system connectome and a hypothesis as to what the circuit is supposed to perform.
We now have a computational method for turning measurements of the connectome into predictions of neural activity and brain function, without first starting with difficult-to-acquire measurements of neural activity for every neuron.
Srini Turaga
“We now have a computational method for turning measurements of the connectome into predictions of neural activity and brain function, without first starting with difficult-to-acquire measurements of neural activity for every neuron,” says Janelia Group Leader Srini Turaga, a senior author on the new research.
A team of scientists from HHMI’s Janelia Research Campus and the University of Tübingen used the connectome to create a precise deep mechanistic network simulation of the fly visual system, with each neuron and synapse in the model representing a real neuron and synapse in the brain. Although the scientists did not know the dynamics of each neuron and synapse, data from the connectome enabled them to estimate these unknown parameters using deep learning approaches. They paired this information with knowledge of the circuit’s purpose: motion detection.
“At that point, everything fell into place, and we could finally figure out if this connectome-constrained model gives us a good model of the brain,” says Janne Lappalainen, a PhD student at the University of Tübingen who led the research.
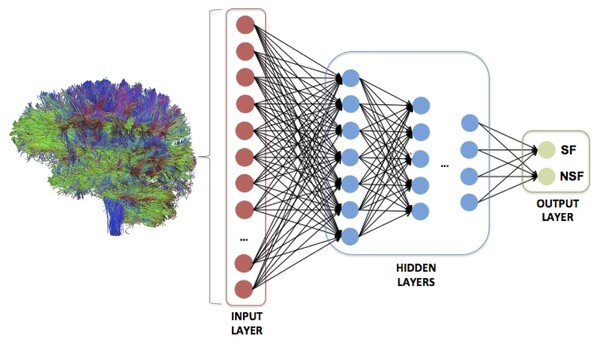
The new model accurately predicts the neuronal activity produced by 64 neuron types in the fruit fly visual system in response to visual input, and it replicates over two dozen experimental experiments conducted over the last two decades.
The new discovery, which allows researchers to predict the activity of individual neurons using only the connectome, has the potential to alter how neuroscientists formulate and test hypotheses about how the brain functions. In theory, scientists may now use the model to simulate any experiment and generate precise predictions that can be tested in the lab.
The new study includes almost 450 pages of predictions derived from the new model, including the identification of cells previously unknown to be involved in motion detection that can now be tested in actual flies.
According to the researchers, the group’s study provides a framework for transforming Janelia and other academic institutes’ vast amounts of connectome data into advanced understanding of the living brain.
“There is a big gap between the static snapshot of the connectome and the dynamics of real-life computation in the living brain, and the question was, can we bridge that gap in a model? This paper, for the specific example of the fruit fly, shows a strategy for bridging that gap,” says Jakob Macke, a senior author on the paper and a professor at the University of Tübingen.