AI has been increasingly used in exoplanet research in recent years. Machine learning algorithms have proven to be very effective in analyzing large amounts of data generated by exoplanet surveys, such as the data from the Kepler space telescope. These algorithms can identify exoplanet signals in the data that might have been missed by traditional analysis methods.
According to new research from the University of Georgia, artificial intelligence can be used to find planets outside our solar system. A recent study demonstrated that machine learning can be used to find exoplanets, which could change the way scientists detect and identify new planets far from Earth.
“One of the novel aspects of this is analyzing environments where planets are still forming,” said Jason Terry, lead author of the study and doctoral student in the UGA Franklin College of Arts and Sciences department of physics and astronomy. “Previously, machine learning was rarely applied to the type of data we’re using, particularly when looking at systems that are still actively forming planets.”
This is a very exciting proof of concept. The power here is that we used exclusively synthetic telescope data generated by computer simulations to train this AI, and then applied it to real telescope data.
Cassandra Hall
The first exoplanet was discovered in 1992, and while over 5,000 are known to exist, those were among the easiest for scientists to discover. Exoplanets in their early stages are difficult to detect for two reasons. They are too far away, often hundreds of light-years from Earth, and the discs that form around them are very thick, thicker than the distance between the Earth and the sun. According to the data, planets tend to be in the center of these discs, leaving a signature of dust and gases kicked up by the planet.
The study demonstrated that artificial intelligence can assist scientists in overcoming these challenges.
“This is a very exciting proof of concept,” said Cassandra Hall, assistant professor of astrophysics, principal investigator of the Exoplanet and Planet Formation Research Group, and co-author on the study. “The power here is that we used exclusively synthetic telescope data generated by computer simulations to train this AI, and then applied it to real telescope data. This has never been done before in our field, and paves the way for a deluge of discoveries as James Webb Telescope data rolls in.”
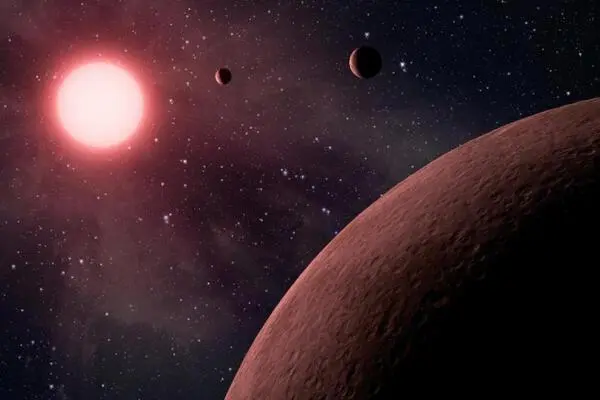
NASA’s James Webb Space Telescope, which was launched in 2021, has ushered in a new era of infrared astronomy, bringing stunning new images and reams of data for scientists to analyze. It’s just the latest iteration of the agency’s search for exoplanets, which are scattered all over the galaxy. The Nancy Grace Roman Observatory, a 2.4-meter survey telescope that will look for dark energy and exoplanets and is scheduled to launch in 2027, will be the next major expansion in capability and data delivery – to comb the universe for life.
The Webb telescope allows scientists to observe exoplanetary systems in extremely bright, high-resolution images, with the forming environments themselves being of great interest as they determine the resulting solar system.
“The potential for good data is exploding, so it’s a very exciting time for the field,” Terry said.
New analytical tools are essential
Next-generation analytical tools are desperately needed to deal with this high-quality data so that scientists can spend more time on theoretical interpretations rather than meticulously combing through the data looking for tiny little signatures.
“In a way, we’ve just made a better person,” Terry explained. “To a large extent, we analyze this data by looking through dozens, hundreds of images for a specific disc and asking ‘is that a wiggle?’ then running a dozen simulations to see if that’s a wiggle and… it’s easy to overlook them — they’re really tiny, and it depends on the cleaning, and so this method is one, really fast, and two, its accuracy gets planets that humans would miss.”
Terry says this is what machine learning can already accomplish – improve on human capacity to save time and money as well as efficiently guide scientific time, investments, and new proposals.
“There is still skepticism about machine learning and AI in science, particularly astronomy, a valid criticism of it being this black box — where you have hundreds of millions of parameters and somehow you get an answer. But we believe we’ve shown in this work that machine learning is capable of the job. You can debate interpretation. In this case, however, we have very concrete results that demonstrate the effectiveness of this method.”
The work of the research team is intended to lay a solid foundation for future applications on observational data by demonstrating the method’s effectiveness through simulational observations.