Random Forest can flexibly incorporate missing data in the predictor variables. When missing data are encountered for a particular observation during model building, the prediction made for that case is based on the last preceding node in the respective tree. Random Forest consists of a collection or ensemble of simple tree predictors, each capable of producing a response when presented with a set of predictor values. It is a notion of the general technique of random decision forests that are an ensemble learning method for classification, regression and other tasks, that operate by constructing a multitude of decision trees at training time and outputting the class that is the mode of the classes or mean prediction of the individual trees.
Random Forest
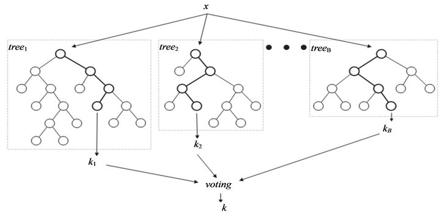