A wearable dataset for predicting in-class exam performance would likely include data from wearable devices such as smartwatches or fitness trackers that capture physiological information about the wearer during the exam period. This might include:
- Heart rate data: to monitor stress levels and indicate periods of high focus or anxiety.
- Activity level data: to determine if the student is restless or distracted during the exam.
- Sleep data: to assess the impact of poor sleep on exam performance.
- Environmental data: temperature, light exposure, etc.
Researchers conducted an experiment in which they collected a set of physiological data from students over the course of three exams. They collected multimodal physiological data with a smartwatch-like wearable device. The smartwatch-like wearable device was used to provide a seamless data collection experience for the students participating in the experiment.
Stress has a negative impact on physical health, lowers work productivity, and results in significant annual costs for industries and healthcare. While high stress is known to increase the risk of cardiovascular disease and have negative effects on mental health, it also has significant effects on one’s ability to complete tasks under either excessively high or excessively low stress. There has been growing research interest on understanding how real-world stress impacts our body and performance, at work and across life activities.
Researchers conducted an experiment in which they collected a set of physiological data from students over the course of three exams. They collected multimodal physiological data with a smartwatch-like wearable device.
Attempts to simulate their impact in the laboratory or elsewhere, however, are less useful than datasets gathered in real-world situations. As a result, researchers have fewer real-world stress datasets to work with. Such datasets are even more uncommon when used in longitudinal studies on the same subjects over time.
Real-world situations are unrestricted environments as well. Access to research-grade equipment is frequently restricted, and motion artifact contamination is widespread. These are still some of the most significant barriers to automated emotion decoders outside of research labs.
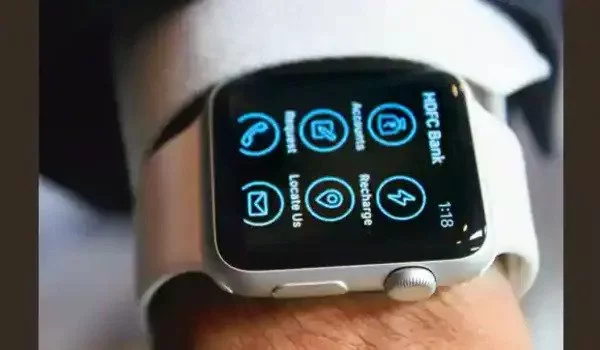
To address the aforementioned gap, Rose Faghih and her former Ph.D. students Md. Rafiul Amin and Dilranjan Wickramasuriya conducted an experiment in which physiological data from students was collected over the course of three exams. They collected multimodal physiological data using a smartwatch-like wearable device. The smartwatch-like wearable device was used to provide students participating in the experiment with a seamless data collection experience.
The investigation shows that it is possible to link the variations in the physiological signals to the exam performance. More details about this study can be found in the corresponding publication titled “A Wearable Exam Stress Dataset for Predicting Grades using Physiological Signals.”
To enable other researchers, use this dataset for additional investigations, the research team has made the de-identified data publicly available on the PhysioNet platform. A Wearable Exam Stress Dataset for Predicting Cognitive Performance in Real-World Settings is available at: https://physionet.org/content/wearable-exam-stress
Finally, the researchers believe it would be extremely beneficial to consider how exam performance and the stress that comes with it interact. It will enable a wide range of potential applications with the goal of improving personal performance. This could help scientists develop effective interventions to improve each individual’s performance and increase productivity within a company, for example. Additionally, the knowledge can be applied in online and remote learning contexts to effectively connect with students and improve learning outcomes.