According to a new study, network science can be utilized to eliminate race and gender prejudice in clinical settings. The findings imply that maintaining clinician peer networks is an effective new strategy to provide safer, more equal health care for women and minorities. Clinical practice bias, particularly bias based on race and gender, is a persistent driver of healthcare inequities. Within an experimental scenario, we evaluated the possibility of a peer-network method to eliminate prejudice in medical treatment decisions.
A study published in Nature Communications by the University of Pennsylvania provides compelling evidence that network science can be utilized to eliminate race and gender bias in clinical settings. The study, led by Professor Damon Centola of the Annenberg School for Communication and the School of Engineering and Applied Science, proposes a novel approach to ensuring safer, more equal health care for women and minorities by regulating clinician peer networks.
Using an experimental methodology, researchers demonstrated that clinicians who initially displayed considerable race and gender bias in their treatment of a clinical case could be persuaded to adjust their therapeutic advice to be bias-free.
“We discovered that by changing the structure of clinicians’ information-sharing networks, we could change doctors’ biased perceptions of their patients’ clinical information,” says Centola, who also directs the Annenberg School’s Network Dynamics Group and is a Senior Fellow of Health Economics at the Leonard Davis Institute. “To put it simply, doctors tend to think differently in networks than they do alone.”
We generally conceive of doctors making reasonable decisions based on medical data, but medical bias is frequently entrenched in professional conventions. Changing the networks of practitioners can alter these norms, resulting in higher-quality treatment suggestions for minority patients.
Professor Damon Centola
The researchers asked 840 professionals to watch a video of a patient delivering a clinical history identifying risk factors for heart disease as part of the trial. The patient was portrayed by a white male actor for half of the physicians and a Black female actor for the other half. Aside from that, the videos were identical.
Based on the patient’s information, clinicians chose one of four treatment recommendations: a dangerous alternative, an under-treatment option, the correct guideline-recommended option, or an over-treatment option.
According to preliminary findings, a Black female patient was 49 percent more likely than a white male patient to be discharged. The white male patient, on the other hand, was 78% more likely than the Black female patient to be referred to the emergency department. The findings confirmed a well-documented fact about American health care: widespread racial and gender bias in medicine.
To counteract these effects, the doctors were divided into two groups: experimental and control. The control groups saw the movie alone, with no interaction from other participants, and had the option to amend their recommendations. The medical bias in the control groups remained unchanged.
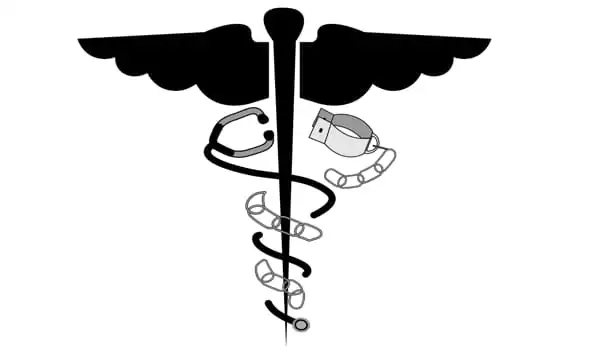
In the experimental scenario, the physicians were linked into huge, anonymous peer networks with 40 other clinicians. Each participant was allowed to see the judgments given by their network peers and had the option to amend their recommendations.
The peer network impacts were astounding. Not only did the networks increase clinical accuracy, but they also removed treatment inequities from physicians’ recommendations, resulting in white male and black female patients obtaining guideline-recommended care at the same rate.
“We generally conceive of doctors making reasonable decisions based on medical data,” Centola says, “but medical bias is frequently entrenched in professional conventions.” Changing the networks of practitioners can alter these norms, resulting in higher-quality treatment suggestions for minority patients.”
Surprisingly, the studies also demonstrated that the network approach to bias reduction increased overall care quality. Researchers discovered that the rate of over-treatment – such as prescribing an unneeded, invasive operation – increased for both patients in the control groups, but fell dramatically for both patients in the experimental groups.
The increasing use of telemedicine and online clinical support networks provide a great opportunity for new information-sharing technologies to aid clinical decision-making. This network-based strategy to removing bias could be used in other medical situations where there are known to be large racial and gender inequities, such as childbirth and acute pain management, as well as mental health and urgent care decisions for COVID-19-related illness.
“The future of medicine is using network technology to improve health care,” Centola argues. “Our next step will be to collaborate with hospital systems around the country to create effective peer-networking initiatives.”
Recent nonclinical research has shown that information transmission in large social networks with uniform—that is, egalitarian—connectivity can be useful for boosting collective intelligence in both health-related and non-health-related risk evaluations. According to research on bias reduction in partisan networks, this process of collective learning in egalitarian networks can successfully diminish, and even eradicate, preexisting political prejudices in the appraisal of novel information.