According to a new study, systems powered by next-generation computer techniques could result in better and more efficient machine learning solutions. Researchers used machine learning technologies to create a digital twin, or virtual replica, of an electronic circuit that exhibits chaotic behavior and were effective in anticipating how it would act and controlling it.
Many daily devices, such as thermostats and cruise control, rely on linear controllers, which apply basic rules to guide a system to a desired value. Thermostats, for example, use such rules to calculate how much to heat or cool a room based on the difference between present and intended temperatures. However, because these algorithms are simple, they struggle to regulate systems with complicated behavior, such as chaos.
As a result, modern technologies such as self-driving cars and aircraft frequently rely on machine learning-based controllers, which employ complex networks to learn the optimal control algorithm required to operate well. However, these algorithms have considerable disadvantages, the most pressing of which is that they can be highly difficult and computationally expensive to implement.
The great thing about the machine learning architecture we used is that it’s very good at learning the behavior of systems that evolve in time. It’s inspired by how connections spark in the human brain.
Robert Kent
Now, having access to an efficient digital twin is expected to have a far-reaching impact on how scientists create future autonomous technology, according to Robert Kent, the study’s senior author and a PhD student in physics at Ohio State University.
“The problem with most machine learning-based controllers is that they use a lot of energy or power and they take a long time to evaluate,” Kent stated. “Developing traditional controllers for them has also been difficult because chaotic systems are extremely sensitive to small changes.”
These concerns, he explained, are essential in instances where milliseconds can mean the difference between life and death, such as when self-driving cars must decide whether to brake to avoid an accident. The findings were just published in Nature Communications.
Compact enough to fit on an inexpensive computer chip capable of balancing on your fingertip and able to run without an internet connection, the team’s digital twin was built to optimize a controller’s efficiency and performance, which researchers found resulted in a reduction of power consumption. It achieves this quite easily, mainly because it was trained using a type of machine learning approach called reservoir computing.
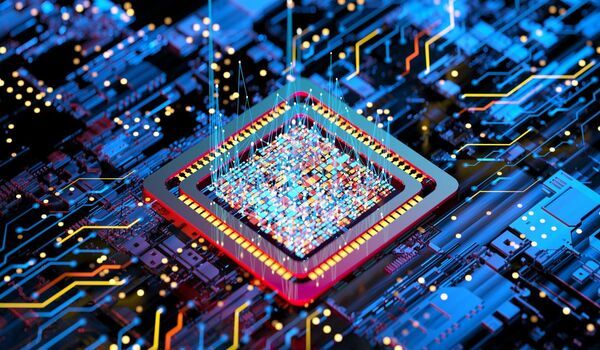
“The great thing about the machine learning architecture we used is that it’s very good at learning the behavior of systems that evolve in time,” Kent said. “It’s inspired by how connections spark in the human brain.”
Although similarly sized computer chips have been used in devices like smart fridges, according to the study, this novel computing ability makes the new model especially well-equipped to handle dynamic systems such as self-driving vehicles as well as heart monitors, which must be able to quickly adapt to a patient’s heartbeat.
“Big machine learning models have to consume lots of power to crunch data and come out with the right parameters, whereas our model and training is so extremely simple that you could have systems learning on the fly,” he said.
To put this hypothesis to the test, researchers gave their model complex control tasks and compared the outcomes to those of earlier control systems. The study found that their strategy outperformed its linear counterpart in terms of task accuracy while being much less computationally complex than a previous machine learning-based controller.
“The increase in accuracy was pretty significant in some cases,” Kent stated. Though the results revealed that their method requires more energy to function than a linear controller, this trade-off means that when powered on, the team’s model lasts longer and is far more efficient than current machine learning-based controllers on the market.
“People will find good use out of it just based on how efficient it is,” Kent said. “You can implement it on pretty much any platform and it’s very simple to understand.” The algorithm was recently made available to scientists.
Outside of inspiring potential advances in engineering, there’s also an equally important economic and environmental incentive for creating more power-friendly algorithms, said Kent.
As society becomes more reliant on computers and artificial intelligence in almost every aspect of daily life, demand for data centers is skyrocketing, prompting many experts to express concern about digital systems’ massive power intake and what future sectors will need to do to stay up.
And, because the construction of these data centers and large-scale computer research can have a significant carbon footprint, scientists are exploring for ways to reduce carbon emissions from this technology.
To improve their findings, future study will most likely focus on training the model to explore other applications such as quantum information processing, Kent explained. Meanwhile, he anticipates that these new aspects will have a broad impact on the scientific community.