According to new Cornell research based on the largest dataset ever used in this area, machine learning can assess the effectiveness of mathematical tools used to predict the movements of financial markets. The researchers’ model could also forecast future market movements, which is an extremely difficult task given the massive amounts of information in markets and their high volatility.
“We were attempting to bring the power of machine learning techniques to not only evaluate how well our current methods and models work, but also to help us extend these in ways that we could never do without machine learning,” said Maureen O’Hara, the Robert W. Purcell Professor of Management at the SC Johnson College of Business.
O’Hara is a co-author of “Microstructure in the Machine Age,” which was published in The Review of Financial Studies.
“Because the databases are so large, estimating these things using standard techniques becomes extremely difficult. The beauty of machine learning is that it provides a new way to analyze data” O’Hara stated. “The main point of this paper is that in some cases, the microstructure features attached to one contract are so powerful that they can predict the movements of other contracts. So we can detect patterns in how markets affect one another, which is extremely difficult to do with standard tools.”
We were attempting to bring the power of machine learning techniques to not only evaluate how well our current methods and models work, but also to help us extend these in ways that we could never do without machine learning.
Maureen O’Hara
Markets generate massive amounts of data, and billions of dollars are at stake in mining that data for patterns that can predict market behavior in the future. Companies on Wall Street and elsewhere use various algorithms to find such patterns and predict the future by examining various variables and factors.
The researchers used a random forest machine learning algorithm in the study to better understand the effectiveness of some of these models. They evaluated the tools using a dataset of 87 futures contracts, which are agreements to buy or sell assets at predetermined prices in the future.
“Our sample is basically all active futures contracts around the world for five years, and we use every single trade – tens of millions of them – in our analysis,” O’Hara said. “What we did is use machine learning to try to understand how well microstructure tools developed for less complex market settings work to predict the future price process both within a contract and then collectively across contracts. We find that some of the variables work very, very well – and some of them not so great.”
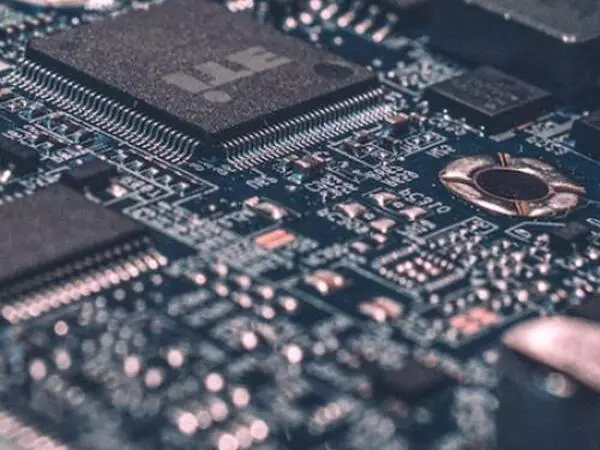
Machine learning has long been used in finance, but typically as a “black box,” in which an artificial intelligence algorithm uses massive amounts of data to predict future patterns without revealing how it does so. This method, according to O’Hara, can be effective in the short term but sheds little light on what actually causes market patterns.
“Our application of machine learning is this: I have a theory about what moves markets; how can I test it?” she explained. “How can I determine whether my theories are sound? And how can I apply what I’ve learned from this machine learning approach to help me build better models and understand things that are too complex to model?”
Huge amounts of historical market data are available — every trade has been recorded since the 1980’s — and vast volumes of information are generated every day. Increased computing power and greater availability of data have made it possible to perform more fine-grained and comprehensive analyses, but these datasets, and the computing power needed to analyze them, can be prohibitively expensive for scholars.
In this study, practitioners from the finance industry collaborated with academic researchers to provide data and computers for the study, as well as expertise in machine learning algorithms used in practice.
“This collaboration benefits both,” said O’Hara, who added that the paper is part of a series of studies she, Easley, and Lopez de Prado have completed over the last decade. “It enables us to conduct research in ways that academic researchers are not normally able to.”