Robots can help advance the development and utilization of solar energy in a variety of ways. Mapping and surveying: Potential solar energy sites can be mapped and surveyed using robots equipped with sensors and imaging technology. They can assess the topography, sunlight exposure, and other relevant factors to determine the viability of solar panel installation.
Researchers created an automated system to perform laboratory experiments and used machine learning to help analyze the data they collected in order to quickly and accurately characterize prospective materials for use in solar energy. Their goal is to find semiconductor materials that are both highly efficient and non-toxic for use in photovoltaic solar energy.
Solar energy is one of the most promising methods of powering the future world. However, developing more efficient solar cells necessitates the discovery of new and improved materials. In a recent study published in JACS Au, researchers at Osaka University unveiled a solution: a system that automates key experimental and analytical processes, significantly speeding up research on solar materials.
Current solar cells are made of inorganic semiconductors containing silicon and gallium, but next-generation solar cells need to reduce both cost and weight. Safety is also a concern; perovskite solar cells are efficient enough to rival silicon solar cells, but they contain toxic lead.
Chisato Nishikawa
Solar cells are made of more than just silicon, and other potential materials may be even more effective. However, in order to be used on a large scale, these materials must meet a few important criteria: they must be highly efficient, made from common chemical elements, and have low toxicity. So far, there aren’t many candidates who can check all three of these boxes. Furthermore, studies into new materials are currently done by hand and are costly and time-consuming.
To speed up the discovery of these materials, the researchers created a unique robotic measurement system that’s able to perform photoabsorption spectroscopy, optical microscopy, and time-resolved microwave conductivity analyses. They then used the robot to evaluate 576 different thin-film semiconductor samples.
“Current solar cells are made of inorganic semiconductors containing silicon and gallium, but next-generation solar cells need to reduce both cost and weight,” explains lead author Chisato Nishikawa. “Safety is also a concern; perovskite solar cells are efficient enough to rival silicon solar cells, but they contain toxic lead.”
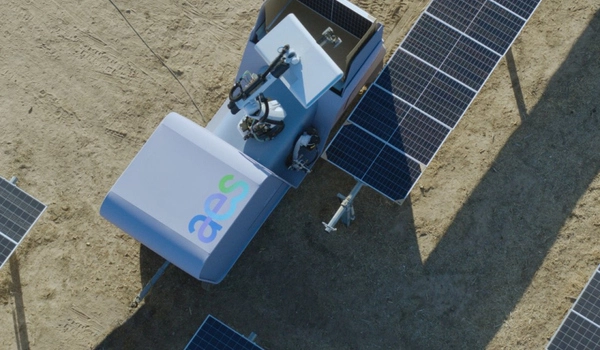
The samples used in this study were all made of a different combination of cesium, bismuth, tin, and iodine. They were also annealed at various temperatures and with various organic salt additives. The researchers examined the data using artificial intelligence, specifically machine learning, to thoroughly characterize the material properties as well as automate the experimental process.
“In recent years, machine learning has been extremely beneficial in better understanding material properties.” These studies necessitate massive amounts of experimental data, and combining automated experiments with machine-learning techniques is a perfect solution,” says senior author Akinori Saeki.
In the future, the authors hope to automate even more of the process, making it easier to examine completely new materials. “This method is ideal for exploring areas where there is no existing data,” Nishikawa says.
So far, the research team’s robotic system has yielded promising results. Because the measurement process is fully automated and highly accurate, work can be completed in one-sixth of the usual time.
The automated system greatly simplifies the task of locating efficient and non-toxic solar materials. Solar energy’s future may be closer than we realize, thanks to robots and artificial intelligence.