Mathematicians developed a statistical model that can detect misinformation in social media posts. A math professor at American University and his colleagues developed a statistical model that can be used to detect misinformation in social media posts. In addition, the model avoids the problem of black boxes that occurs in machine learning.
Machine learning is increasingly being used to help stop the spread of misinformation through the use of algorithms and computer models, but a major challenge for scientists is the black box of unknowability, in which researchers don’t understand how the machine arrives at the same decision as human trainers.
Using a Twitter dataset containing misinformation tweets about COVID-19, Zois Boukouvalas, assistant professor in the Department of Mathematics and Statistics, College of Arts and Sciences at AU, demonstrates how statistical models can detect misinformation in social media during events such as a pandemic or natural disaster. Boukouvalas and his colleagues, including AU student Caitlin Moroney and Computer Science Prof. Nathalie Japkowicz, show how the model’s decisions align with those made by humans in newly published research.
What’s significant about this finding is that our model achieved accuracy while also providing transparency about how it detected misinformation in tweets. Deep learning methods cannot achieve this level of accuracy while remaining transparent.
Zois Boukouvalas
“We’d like to know what a machine is thinking when it makes decisions, as well as how and why it agrees with the humans who trained it,” Boukouvalas explained. “We don’t want to block someone’s social media account because the model makes an erroneous decision.”
Boukouvalas’ method is a type of statistical machine learning. It is not as well-known as deep learning, which is a complex, multi-layered type of machine learning and artificial intelligence. Statistical models are effective and provide another, largely untapped, an avenue for combating misinformation, according to Boukouvalas.
The model achieved a high prediction performance and correctly classified 112 real and misinformation tweets in a testing set of 112 tweets, with an accuracy of nearly 90%. (Using such a small dataset was a quick way to see how the method detected misinformation tweets.)
“What’s significant about this finding is that our model achieved accuracy while also providing transparency about how it detected misinformation in tweets,” Boukouvalas added. “Deep learning methods cannot achieve this level of accuracy while remaining transparent.”
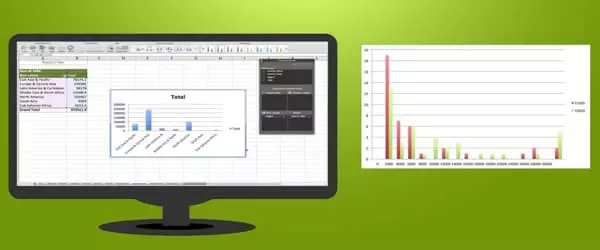
Researchers first prepared to train the model before testing it on the dataset. Models are only as good as the information provided by humans. Human biases are introduced (which is one of the causes of bias in facial recognition technology), and black boxes are created.
The tweets were carefully labeled as either misinformation or real by the researchers, who used a set of pre-defined rules about the language used in misinformation to guide their decisions. They also considered human language nuances and linguistic features associated with misinformation, such as a post that makes extensive use of proper nouns, punctuation, and special characters. Prof. Christine Mallinson of the University of Maryland Baltimore County, a socio-linguist, identified the tweets for writing styles associated with misinformation, bias, and less trustworthy sources in news media. It was then time to train the model.
“Once we put those inputs into the model, it tries to understand the underlying factors that lead to the separation of good and bad information,” Japkowicz explained. “It’s about understanding the context and how words interact.”
For example, in two of the tweets in the dataset, the words “bat soup” and “covid” appear together. The researchers labeled the tweets as misinformation, and the model identified them as such. The model identified hate speech, hyperbolic language, and strongly emotional language in the tweets, all of which are associated with misinformation. This implies that the model recognized the human decision behind the labeling in each of these tweets and followed the researchers’ rules.
The model’s user interface will be improved next, as will the model’s ability to detect misinformation in social posts that include images or other multimedia. The statistical model must learn how various elements in social posts interact to create misinformation. In its current form, the model is best suited for use by social scientists or other researching methods to detect misinformation.
Despite advances in machine learning to aid in the fight against misinformation, Boukouvalas and Japkowicz agreed that human intelligence and news literacy remain the first lines of defense in preventing misinformation from spreading.
“Through our work, we design machine learning-based tools to alert and educate the public in order to eliminate misinformation, but we strongly believe that humans must play an active role in preventing misinformation from spreading in the first place,” Boukouvalas said.