The ability of AI to grasp similar concepts after learning one concept is determined by the individual AI model, training data, and learning strategies employed. Because they are trained on enormous volumes of text data and can capture multiple links between words and concepts, AI models like GPT-3 and its descendants can grasp and develop related concepts after learning one notion.
Researchers have now created an approach that improves these tools’ ability to make compositional generalizations, such as ChatGPT. This technique, Meta-learning for Compositionality, surpasses existing approaches and performs on par with, if not better than, human performance in some circumstances.
Humans have the ability to learn a new notion and then quickly apply it to grasp related applications of that concept; for example, once youngsters understand how to “skip,” they understand what it means to “skip twice around the room” or “skip with your hands up.”
Is it possible for machines to think like this? In the late 1980s, philosophers and cognitive scientists Jerry Fodor and Zenon Pylyshyn argued that artificial neural networks, the engines that power artificial intelligence and machine learning, are incapable of forming these connections, known as “compositional generalizations.” However, in the decades afterward, scientists have been working on ways to implant this capability in neural networks and associated technologies, with varying degrees of success, thereby keeping this decades-old argument alive.
Large language models, such as ChatGPT, still struggle with compositional generalization, though they have gotten better in recent years. However, we think that MLC can further improve the compositional skills of large language models.
Marco Baroni
Researchers at New York University and Spain’s Pompeu Fabra University have now developed a technique – reported in the journal Nature – that advances the ability of these tools, such as ChatGPT, to make compositional generalizations. This technique, Meta-learning for Compositionality (MLC), outperforms existing approaches and is on par with, and in some cases better than, human performance. MLC centers on training neural networks – the engines driving ChatGPT and related technologies for speech recognition and natural language processing – to become better at compositional generalization through practice.
Existing system developers, particularly huge language models, have either hoped for compositional generalization to emerge from ordinary training methods or have created special-purpose structures to attain these skills. According to the authors, MLC demonstrates how actively honing these abilities allows these systems to unlock additional powers.
“For the past 35 years, researchers in cognitive science, artificial intelligence, linguistics, and philosophy have been debating whether neural networks can achieve human-like systematic generalization,” says Brenden Lake, an assistant professor in NYU’s Center for Data Science and Department of Psychology and one of the paper’s authors. “We have shown, for the first time, that a generic neural network can mimic or exceed human systematic generalization in a head-to-head comparison.”
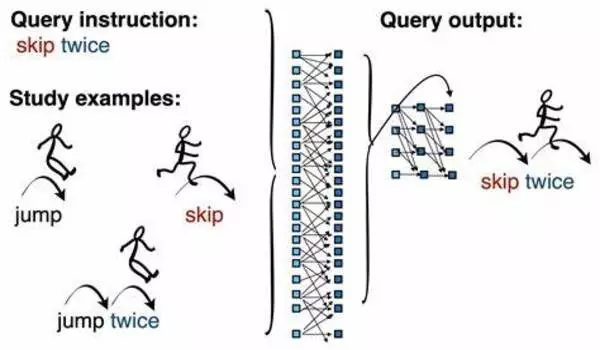
In order to investigate the possibilities of enhancing compositional learning in neural networks, the researchers developed MLC, a unique learning technique in which a neural network’s skills are continually updated across a series of episodes. In an episode, MLC is given a new word and instructed to utilize it in a compositional way, such as taking the word “jump” and then creating new word combinations like “jump twice” or “jump around right twice.” The network then receives a new episode with a different term, and so on, each time strengthening its compositional skills.
To test the effectiveness of MLC, Lake, co-director of NYU’s Minds, Brains, and Machines Initiative, and Marco Baroni, a researcher at the Catalan Institute for Research and Advanced Studies and professor at the Department of Translation and Language Sciences of Pompeu Fabra University, conducted a series of experiments with human participants that were identical to the tasks performed by MLC.
Furthermore, rather than learning the meaning of genuine words – terms that humans are already familiar with – they had to learn the meaning of nonsensical phrases as specified by the researchers and how to apply them in various ways. MLC performed as well as or better than the human participants in some circumstances. MLC and humans also outscored ChatGPT and GPT-4, which, despite their impressive general abilities, struggled with this learning test. “Large language models, such as ChatGPT, still struggle with compositional generalization, though they have gotten better in recent years,” says Baroni, a member of the Pompeu Fabra University’s Computational Linguistics and Linguistic Theory research group. “But we think that MLC can further improve the compositional skills of large language models.”