Network-based technologies are becoming more common, and they are now used by countless individuals, professionals, and businesses all over the world. Regardless of their benefits, most network-based systems are extremely vulnerable to malicious attacks.
A malicious attack on network-based systems can have extremely severe and devastating consequences. An attack on a power utility network, for example, could knock out power to millions of people and businesses, whereas attacks on social media networks could result in breaches of confidential user information.
To address the vulnerabilities of network-based systems, computer scientists around the world have been working to create advanced intrusion detection systems (IDSs) that can help identify and counteract malicious attacks, thereby increasing network security. In recent years, machine learning (ML) algorithms have been found to be particularly promising for automatically detecting network attacks and intrusions.
Network-based technologies have become increasingly widespread, and they are now being used by countless individuals, professionals, and businesses worldwide.
Researchers are working hard to create efficient models with high detection accuracy, which has sparked a lot of interest in intrusion detection. Nonetheless, the challenge remains in developing a dependable and efficient Intrusion Detection System (IDS) capable of handling large amounts of data, with trends changing in real-time conditions. The detection methods used, particularly feature selection techniques and machine learning algorithms, are critical in the design of such a system.
The selection of data features on which a model can rely or focus when making predictions is an important step in the development and training of ML-based IDSs. Ideally, researchers should be able to identify the most suitable features for solving a given task using ML tools by analyzing large datasets, and this is also applicable to intrusion detection.
In intrusion detection, feature selection is an important pre-processing step. Achieving a reduction in the number of relevant traffic features while maintaining classification accuracy is a goal that greatly improves an intrusion detection system’s overall effectiveness. The selection of appropriate feature-selection methods that can precisely determine the relevance of features to the intrusion detection task and the redundancy between features is a major challenge.
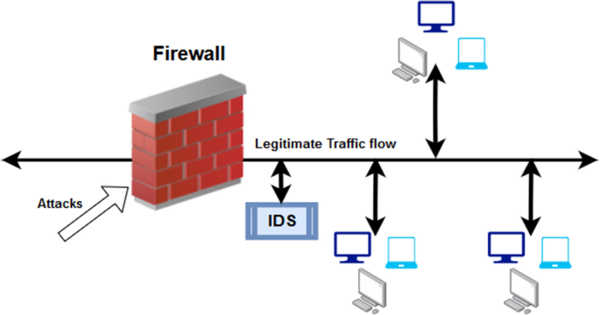
Researchers at Canadian University Dubai in the United Arab Emirates recently developed a new feature selection method that could lead to the development of more effective ML-based intrusion detection systems. When compared to other commonly used feature selection techniques, this method, presented in a paper pre-published on arXiv, performed remarkably well.
“Our goal is to study feature selection in network traffic data with the goal of detecting potential attacks,” the study’s authors, Firuz Kamalov, Sherif Moussa, Rita Zgheib, and Omar Mashaal, wrote in their paper. “We examine existing feature selection methods and propose a new feature selection algorithm to identify the most powerful features in network traffic data.”
First, Kamalov and his colleagues investigated a variety of feature selection methods that could be used to detect features or characteristics of network traffic data relevant to intrusion detection. They concentrated on three standard selection methods: correlation-based univariate, MI-based univariate, and correlation-based forward search algorithms.
Following that, the researchers created MICorr, a new feature selection method that addresses some of the limitations of existing feature selection techniques. They tested this method on the CSE-CIC-IDS2018 dataset, which contains 10,000 instances of benign and malicious network intrusion.
“We propose a new feature selection method that addresses the challenge of taking into account both continuous input features and discrete target values,” the researchers wrote in their paper. “We demonstrate that the proposed method outperforms the benchmark selection methods.”
Kamalov and his colleagues developed a highly efficient ML-based detection system based on the features they identified as important for intrusion detection. This system was found to be 99 percent accurate in distinguishing between DDoS (Distributed Denial of Service) attacks and harmless network signals.
The feature selection method developed by this team of researchers, as well as the findings presented in their paper, could be used to develop new, highly effective IDSs in the future. Furthermore, the system they developed using the features they discovered could be used in real-world settings to detect malicious attacks on real networks.