Most electric vehicles are outfitted with an electronic brain that monitors battery performance and safety on a daily basis. This battery management system, or BMS, incorporates software that monitors the overall health of the powerful lithium-ion battery pack using algorithms.
“The algorithm tells you things like whether your battery is charging properly or how far you can drive before needing to recharge,” said Simona Onori, an assistant professor of energy science and engineering at Stanford’s Doerr School of Sustainability. “The problem is that BMS algorithms are designed in ideal laboratory conditions that do not reflect what a battery pack sees in the real world.”
Onori and colleagues at Stanford cooperated with experts at the Volkswagen Innovation and Engineering Center, which is located near the university campus, to highlight the gap between controlled laboratory testing and actual road experience. Their findings were published in the journal Joule on August 18.
“Algorithms based on unrealistic driving data are likely to be inaccurate in the field,” explained Onori, the study’s lead author. “Our goal is to increase the battery pack’s longevity by designing algorithms trained on real-world data.”
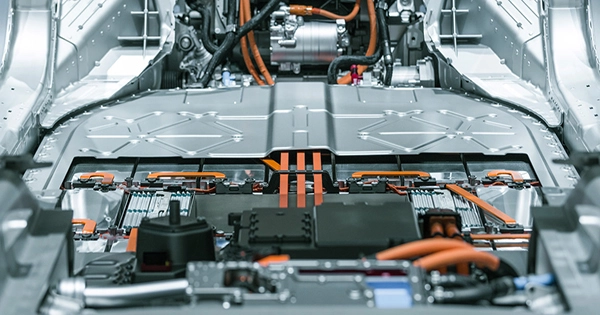
Driving styles: In today’s electric vehicles, battery management systems routinely capture data during braking, acceleration, deceleration, and charging.
“Real-world driving is driver-specific,” said study co-author and Stanford research engineer Gabriele Pozzato. “You may be a reckless driver or someone who only half charges your automobile. varied driving and charging strategies will result in varied battery degradation trajectories. However, traditional battery algorithms do not include this type of field data.”
Volkswagen gave the Stanford team about 3,750 hours of BMS driving data recorded from an all-electric Audi e-tron SUV driven in the San Francisco Bay Area for a year, from November 2019 to October 2020 (Audi is a Volkswagen subsidiary).
Power and Energy: The Stanford scientists calculated the electrical resistance in the battery pack over a one-year period using Audi field data. These computations allowed the researchers to evaluate two important battery metrics: energy and power.
“Energy gives you the range, or how many miles you can drive with a fully charged battery,” added Pozzato. “Power is the ability to quickly extract energy.” When you accelerate, you want to quickly access energy and deplete the battery. The lower the electrical resistance in the battery, the greater the power.”
To determine resistance, the researchers used data from 529 accelerating events and 392 braking events over the course of a year to monitor rapid changes in current and voltage in the battery pack. In addition, they calculated impedance (a measure of resistance during battery charging) by evaluating 53 charging sessions.
“Impedance and resistance are typically considered metrics of battery health,” Onori explained. “The more you drive, the greater the resistance.” This normally results in less accessible power from the battery pack, although this was not the case for us.”
When the researchers added seasonal meteorological data to the mix, a more complex pattern formed. They noticed that electrical resistance reduced in the cooler months and grew consistently in the spring and summer, indicating that battery health improves as temperatures rise.
“Higher temperatures increase battery capacity, giving you the impression that the car has more energy and that you can drive farther,” Onori explained. “However, if you continue to use the battery at high temperatures, it will degrade faster.” These are tricky aspects that influence performance. Next year, we’ll expand our dataset to include a fleet of automobiles to better understand how temperature and age interact.”
The laboratory versus the road: Automobile manufacturers rely on traditional BMS algorithms developed in pristine laboratory circumstances. These methods typically use machine learning to monitor performance data from a single 4-volt battery cell that continuously charges and discharges at a fixed temperature until it dies. The Audi field data, on the other hand, was gathered from a 396-volt battery pack powered by 384 cells.
“New algorithms should focus on the entire battery pack rather than individual cells,” stated Onori. “We want to create algorithms that educate drivers on how to extend the life of the vehicle’s battery pack, which is the most expensive component.” For example, you may warn drivers if they are charging too quickly or accelerating too quickly. Field data can teach us so much about how to improve the robustness of BMS algorithms.”