The removal of soil along drainage lines by surface water runoff is known as gully erosion. Gullies will continue to move by headward erosion or slumping of the side walls unless steps are taken to stabilize the disturbance. Repair work is far easier and less expensive in the early stages of newly formed gullies. Large gullies that have grown unchecked are difficult and expensive to repair.
Soil erosion is a major issue for agricultural production, affecting soil quality and allowing pollutants into waterways. Gully erosion is the most severe stage of soil erosion, with large channels carved through the field. Once gullies form, they are difficult to manage with tiling; they necessitate a more comprehensive approach along the impacted area. Researchers have now created a modeling framework that uses remote sensing environmental data to accurately predict gully erosion susceptibility. Landowners and conservation organizations can use this predictive model to direct management resources to the most vulnerable areas.
Soil erosion is a major issue for agricultural production, affecting soil quality and allowing pollutants into waterways. Gully erosion is the most severe stage of soil erosion, with large channels carved through the field. Once gullies form, they are difficult to manage with tiling; they necessitate a more comprehensive approach along the impacted area.
Researchers at the University of Illinois created a modeling framework that uses remote sensing environmental data to accurately predict gully erosion susceptibility. Landowners and conservation organizations can use this predictive model to direct management resources to the most vulnerable areas.
We predict the geospatial location of gully erosion using high-resolution spatial and temporal data from satellite sensing. We used the maximum entropy model, or MaxEnt, to predict areas with a high probability of gully erosion.
Jeongho Han
“Erosion processes are complicated to predict, because there are so many factors at play, including farmer activity, climate, rainfall, temperature, vegetation development, topography, and many other variables that are always changing over time. We wanted to incorporate more of these variabilities in space and time in our model to decrease the uncertainty of the prediction,” says Jorge Guzman, research assistant professor in the Department of Agricultural and Biological Engineering (ABE) at the U of I and co-author on the paper, published in the Journal of Hydrology: Regional Studies.
The study was carried out in Jefferson County, Illinois, where agricultural production accounts for 59% of the land use, primarily corn and soybeans. The region is typical of Midwest row crop production.
“We predict the geospatial location of gully erosion using high-resolution spatial and temporal data from satellite sensing,” says Jeongho Han, the paper’s lead author and doctoral student in ABE.
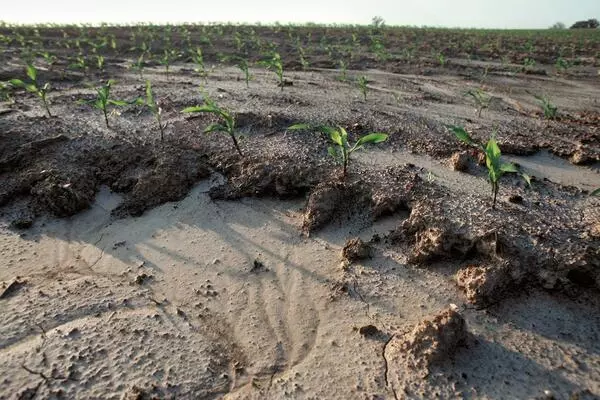
“We used the maximum entropy model, or MaxEnt, to predict areas with a high probability of gully erosion. Typically, researchers have focused on static variables like soil, elevation, and slope, but we added temporal variables like precipitation and vegetation because erosion is highly affected by crop growth, temperature, and rainfall intensity,” Han says.
“For example, Illinois has a bimodal rain pattern, with heavier rainfall during the spring and fall seasons. We need to consider the temporal variability of these factors.”
The addition of dynamic variables aided the researchers in developing a modeling framework that more accurately represents the complexity of factors influencing erosion.
Han and Guzman analyzed LiDAR data from the Illinois Geospatial Data Clearinghouse mapped at 2-meter spatial resolution, which provides airborne surface light detection for all of Illinois, to confirm their modeling results with actual gully locations. They were able to identify changes in surface elevation that could indicate the formation of gullies by comparing images from two different years. These identified locations were then filtered and processed to remove direct human intervention such as mining, construction, and other activities, as well as to narrow the gully inference to the LiDAR accuracy.
Overall, the researchers found 7.4% of the agricultural land in the study area had an elevated risk of developing gully erosion. Among all factors considered, the slope, land use, seasonal daily maximum precipitation, and organic matter indicated the highest contribution in predicting the presence of gullies. The researchers also found that spatiotemporal changes in land cover and precipitation were crucial in predicting gully formation in agricultural areas.
Their approach is applicable to agricultural areas in the Midwest of the United States that have similar land management and environmental variables.
“The main idea is that if we know where gullies are more likely to form, we can begin to implement land management practices,” Guzman explains. “There are numerous tools and programs available to help with erosion and nutrient management. The challenge is determining how to optimize these efforts. Our tools can help landowners, communities, policymakers, and conservation organizations target programs and processes, directing resources where they are most needed.”