A sensor network is a collection of sensors in which each sensor observes data at a different area and delivers that data to a central location for storage, display, and analysis. Sensor networks have a wide range of uses, from monitoring a single home to monitoring a huge city to detecting earthquakes around the world.
Wireless sensor networks (WSNs) are networks of spatially dispersed and dedicated sensors that monitor and record the physical conditions of the environment and send the acquired data to a central point. WSNs can monitor ambient parameters such as temperature, sound, pollution levels, humidity, and wind.
These are similar to wireless ad hoc networks in that they rely on wireless connectivity and the spontaneous creation of networks to carry sensor data wirelessly. Temperature, sound, and pressure are examples of physical or environmental conditions monitored by WSNs. Modern networks are bidirectional, capable of gathering data as well as controlling sensor activity. Military uses such as battlefield monitoring drove the creation of these networks. Like networks are utilized in industrial and consumer applications such as process monitoring and control, as well as machine health monitoring.
Smiga’s discovery could lead to better vector field measurements, which map the fluctuating magnitudes and orientations of physical quantities in space. This research proposes a new way to quantify the quality of sensor networks, and uses this method to suggest improvements to existing Dark Matter experiments.
When building sensor networks, it can be extremely challenging for researchers to determine how the sensors should be arranged to obtain optimal results. New research proposes a new way to quantify the quality of sensor networks, and uses this method to suggest improvements to existing Dark Matter experiments.
Many studies use multiple sensors in sophisticated networks to collect data rather than a single, centralized sensor. This has a number of advantages, including increased sensitivity and resolution in experimental measurements, as well as the capacity to detect and repair errors more effectively. With all of the complications required in monitoring each sensor and gathering all of their data streams at simultaneously, determining how the sensors should be organized to achieve optimal results can be quite difficult. Joseph Smiga of Johannes Gutenberg University Mainz presents a novel methodology to quantify the quality of sensor networks in new research published in EPJ D, and utilizes his methods to recommend improvements to current trials.
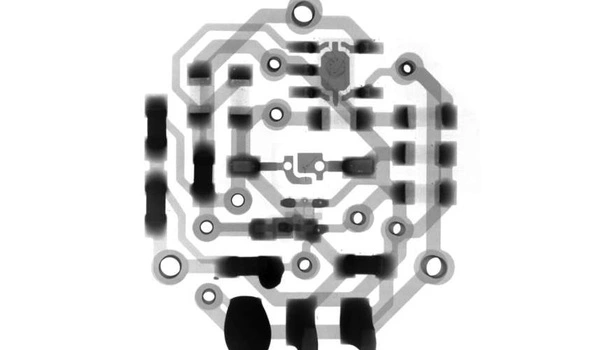
Smiga’s discovery could lead to better vector field measurements, which map the fluctuating magnitudes and orientations of physical quantities in space. Sensor networks are critical in these investigations because they allow researchers to quantify phenomena such as gravitational waves and small shifts in the Earth’s gravitational field. Furthermore, they are currently being utilized in the search for Dark Matter: the enigmatic substance thought to account for a huge part of the universe’s overall mass, but which very weakly interacts with conventional matter, making it infamously difficult to detect directly.
The Global Network of Optical Magnetometers for Exotic Physics Searches (GNOME) is one such experiment, which includes a network of magnetometers spread throughout the globe. The goal of the study is to discover novel, as-yet theoretical vector fields associated with Dark Matter, which link to the quantum spins of protons and neutrons, producing an effect akin to a magnetic field. Smiga offers a method for calculating the sensitivity of a sensor network in his research. This enabled him to measure how well its sensors are grouped and, as a result, to recommend how networks should be optimized. His findings show that by reorienting the sensing orientations of the GNOME network’s existing magnetometers, the sensitivity of the network might be enhanced over prior iterations of the experiment.
Sensor technology has evolved dramatically, from wireless sensor networks (WSN) to the Internet of Things (IoT) (IoT). Existing research on clustering techniques reveals that their focus is more on common concerns than on data redundancy, which should be controlled to improve data quality. In WSN, the closer relationship between redundancy and data quality has received less attention in search of a practical solution.
As a result, we introduce DQRF, or Data Quality Resembles Factor, a framework designed solely for performing clustering operations to improve data quality. Four consecutive techniques are used to discover the node that contains considerable redundant information in the framework. The idea is to capture global details of all the nodes in the short run of clustering to identify and eliminate all possible errors. The proposed system offers approximately 93% of enhancement in data in contrast to the existing system.