Expectation Maximization Algorithm is a natural generalization of maximum likelihood estimation to the incomplete data case. It is used to find maximum likelihood parameters of a statistical model in cases where the equations cannot be solved directly. Typically these models involve latent variables in addition to unknown parameters and known data observations. It has become a popular tool in statistical estimation problems involving incomplete data, or in problems which can be posed in a similar form, such as mixture estimation. Expectation Maximization Algorithm has also been used in various motion estimation frameworks and variants of it have been used in multiframe superresolution restoration methods which combine motion estimation along the lines of.
Expectation Maximization Algorithm
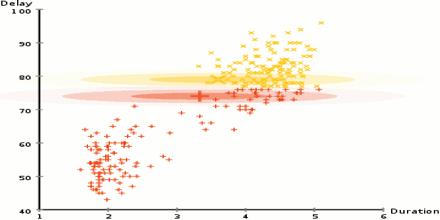