The M87 black hole is in the center of the M87 galaxy, which is 55 million light-years away from Earth. Thanks to the Event Horizon Telescope (EHT) collaboration in April 2019, it was the first black hole to be directly imaged. The image showed a bright, circular ring of light surrounding a dark center, which is the shadow of the event horizon of a black hole.
The iconic image of M87’s supermassive black hole, also known as the “fuzzy, orange donut,” has received its first official makeover thanks to machine learning. The new image reveals a larger and darker central region surrounded by bright accreting gas shaped like a “skinny donut.” The team used data collected by the Event Horizon Telescope (EHT) collaboration in 2017 to achieve full array resolution for the first time.
In 2017, the EHT collaboration used a network of seven pre-existing telescopes located around the world to collect data on M87, resulting in the creation of a “Earth-sized telescope.” However, since it is infeasible to cover the Earth’s entire surface with telescopes, gaps arise in the data – like missing pieces in a jigsaw puzzle.
“With our new machine learning technique, PRIMO, we were able to achieve the maximum resolution of the current array,” says Institute for Advanced Study lead author Lia Medeiros. “Because we can’t study black holes up close, image detail is critical to our understanding of their behavior.” The width of the ring in the image is now about a factor of two smaller, which will be a powerful constraint for our theoretical models and gravity tests.”
PRIMO, which stands for principal-component interferometric modeling, was developed by EHT members Lia Medeiros (Institute for Advanced Study), Dimitrios Psaltis (Georgia Tech), Tod Lauer (NOIRLab), and Feryal Özel (Georgia Tech). Their publication, “The Image of the M87 Black Hole Reconstructed with PRIMO,” is now available in The Astrophysical Journal Letters.
We are using physics to fill in regions of missing data in a way that has never been done before by using machine learning. This could have significant implications for interferometry, which is used in fields ranging from exoplanets to medicine.
Lia Medeiros
“PRIMO is a new approach to the difficult task of constructing images from EHT observations,” said Lauer. “It provides a way to compensate for the missing information about the object being observed, which is required to generate the image that would have been seen using a single gigantic radio telescope the size of the Earth.”
PRIMO is based on dictionary learning, a subset of machine learning that allows computers to generate rules based on large amounts of training data. For example, if a computer is fed a series of different banana images, with enough training, it may be able to determine whether or not an unknown image is a banana. Beyond this simple example, machine learning’s versatility has been demonstrated in a variety of ways, including the creation of Renaissance-style works of art and the completion of Beethoven’s unfinished work. So, how can machines assist scientists in rendering a black hole image? This question has been addressed by the research team.
Over 30,000 high-fidelity simulated images of black holes accreting gas were analyzed using PRIMO. The simulations covered a wide range of models for how a black hole accretes matter, looking for common patterns in the image structure. The various structure patterns were sorted by how frequently they occurred in the simulations and then blended to provide a highly accurate representation of the EHT observations while also providing a high-fidelity estimate of the missing structure of the images.
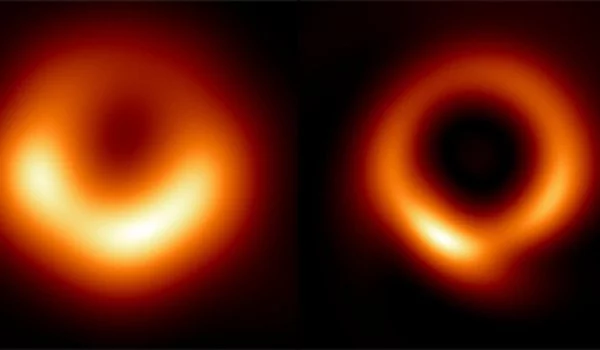
“We are using physics to fill in regions of missing data in a way that has never been done before by using machine learning,” Medeiros explained. “This could have significant implications for interferometry, which is used in fields ranging from exoplanets to medicine.”
The team confirmed that the newly rendered image is consistent with the EHT data and theoretical expectations, including the bright ring of emission that hot gas falling into the black hole is expected to produce. Creating an image required assuming an appropriate form of the missing information, which PRIMO accomplished by building on the 2019 discovery that the M87 black hole looked as predicted in broad detail.
“Approximately four years after the first horizon-scale image of a black hole was unveiled by EHT in 2019, we have marked another milestone, producing an image that utilizes the full resolution of the array for the first time,” stated Psaltis. “The new machine learning techniques that we have developed provide a golden opportunity for our collective work to understand black hole physics.”
The new image should lead to more precise estimates of the M87 black hole’s mass and the physical parameters that determine its current appearance. The data also allows researchers to place more constraints on alternatives to the event horizon (due to the darker central brightness depression) and perform more robust gravity tests (due to the narrower ring size). PRIMO can also be used to analyze additional EHT observations, such as Sgr A*, the central black hole in our own Milky Way galaxy.
M87 is a large, nearby galaxy in the Virgo cluster of galaxies. A mysterious jet of hot plasma was observed to emanate from its center over a century ago. Beginning in the 1950s, the then-new technique of radio astronomy showed the galaxy to have a compact bright radio source at its center. During the 1960s, M87 had been suspected to have a massive black hole at its center powering this activity.
Measurements taken with ground-based telescopes beginning in the 1970s, and later with the Hubble Space Telescope beginning in the 1990s, provided strong evidence that M87 did, in fact, contain a black hole weighing several billion times the mass of the Sun, based on observations of the high velocities of stars and gas orbiting its center. The 2017 EHT observations of M87 were obtained over several days using multiple radio telescopes linked together at the same time to achieve the highest resolution possible. The now-iconic “orange donut” image of the M87 black hole, released in 2019, was the first attempt to create an image based on these observations.
“The 2019 image was just the beginning,” stated Medeiros. “If a picture is worth a thousand words, the data underlying that image have many more stories to tell. PRIMO will continue to be a critical tool in extracting such insights.”